Abstract
Theories of visual narrative comprehension have advocated for a hierarchical grammar-based comprehension mechanism, but only limited work has investigated this hierarchy. Here, we provide a computational framework inspired by computational psycholinguistics to address hierarchy in visual narratives. The predictions generated by this framework were compared against behavior data to draw inferences about the hierarchical properties of visual narratives. A segmentation task—where participants ranked all possible segmental boundaries—demonstrated that participants’ preferences were predicted by visual narrative grammar. Three kinds of models using surprisal theory—an Earley parser, a hidden Markov model (HMM), and an n-gram model—were then used to generate segmentation preferences for the same task. Earley parser’s preferences were based on a hierarchical grammar with recursion properties, while the HMM and the n-grams used a flattened grammar for visual narrative comprehension. Given the differences in the mechanics of these models, contrasting their predictions against behavior data could provide crucial insights into understanding the underlying mechanisms of visual narrative comprehension. By investigating grammatical systems outside of language, this research provides new directions to explore the generic makeup of the cognitive structure of mental representations.
Read Full Article (External Site)
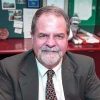
Dr. David Lowemann, M.Sc, Ph.D., is a co-founder of the Institute for the Future of Human Potential, where he leads the charge in pioneering Self-Enhancement Science for the Success of Society. With a keen interest in exploring the untapped potential of the human mind, Dr. Lowemann has dedicated his career to pushing the boundaries of human capabilities and understanding.
Armed with a Master of Science degree and a Ph.D. in his field, Dr. Lowemann has consistently been at the forefront of research and innovation, delving into ways to optimize human performance, cognition, and overall well-being. His work at the Institute revolves around a profound commitment to harnessing cutting-edge science and technology to help individuals lead more fulfilling and intelligent lives.
Dr. Lowemann’s influence extends to the educational platform BetterSmarter.me, where he shares his insights, findings, and personal development strategies with a broader audience. His ongoing mission is shaping the way we perceive and leverage the vast capacities of the human mind, offering invaluable contributions to society’s overall success and collective well-being.