Cognitive scientists often infer multidimensional representations from data. Whether the data involve text, neuroimaging, neural networks, or human judgments, researchers frequently infer and analyze latent representational spaces (i.e., embeddings). However, the properties of a latent representation (e.g., prediction performance, interpretability, compactness) depend on the inference procedure, which can vary widely across endeavors. For example, dimensions are not always globally interpretable and the dimensionality of different embeddings may not be readily comparable. Moreover, the dichotomy between multidimensional spaces and purportedly richer representational formats, such as graph representations, is misleading. We review what the different notions of dimension in cognitive science imply for how these latent representations should be used and interpreted.
Read Full Article (External Site)
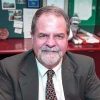
Dr. David Lowemann, M.Sc, Ph.D., is a co-founder of the Institute for the Future of Human Potential, where he leads the charge in pioneering Self-Enhancement Science for the Success of Society. With a keen interest in exploring the untapped potential of the human mind, Dr. Lowemann has dedicated his career to pushing the boundaries of human capabilities and understanding.
Armed with a Master of Science degree and a Ph.D. in his field, Dr. Lowemann has consistently been at the forefront of research and innovation, delving into ways to optimize human performance, cognition, and overall well-being. His work at the Institute revolves around a profound commitment to harnessing cutting-edge science and technology to help individuals lead more fulfilling and intelligent lives.
Dr. Lowemann’s influence extends to the educational platform BetterSmarter.me, where he shares his insights, findings, and personal development strategies with a broader audience. His ongoing mission is shaping the way we perceive and leverage the vast capacities of the human mind, offering invaluable contributions to society’s overall success and collective well-being.