BackgroundParkinson’s disease (PD) is a prevalent neurodegenerative disorder that significantly benefits from early diagnosis for effective disease management and intervention. Despite advancements in medical technology, there remains a critical gap in the early and non-invasive detection of PD. Current diagnostic methods are often invasive, expensive, or late in identifying the disease, leading to missed opportunities for early intervention.ObjectiveThe goal of this study is to explore the efficiency and accuracy of combining fNIRS technology with machine learning algorithms in diagnosing early-stage PD patients and to evaluate the feasibility of this approach in clinical practice.MethodsUsing an ETG-4000 type near-infrared brain function imaging instrument, data was collected from 120 PD patients and 60 healthy controls. This cross-sectional study employed a multi-channel mode to monitor cerebral blood oxygen changes. The collected data were processed using a general linear model and β values were extracted. Subsequently, four types of machine learning models were developed for analysis: Support vector machine (SVM), K-nearest neighbors (K-NN), random forest (RF), and logistic regression (LR). Additionally, SHapley Additive exPlanations (SHAP) technology was applied to enhance model interpretability.ResultsThe SVM model demonstrated higher accuracy in differentiating between PD patients and control group (accuracy of 85%, f1 score of 0.85, and an area under the ROC curve of 0.95). SHAP analysis identified the four most contributory channels (CH) as CH01, CH04, CH05, and CH08.ConclusionThe model based on the SVM algorithm exhibited good diagnostic performance in the early detection of PD patients. Future early diagnosis of PD should focus on the Frontopolar Cortex (FPC) region.
Read Full Article (External Site)
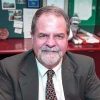
Dr. David Lowemann, M.Sc, Ph.D., is a co-founder of the Institute for the Future of Human Potential, where he leads the charge in pioneering Self-Enhancement Science for the Success of Society. With a keen interest in exploring the untapped potential of the human mind, Dr. Lowemann has dedicated his career to pushing the boundaries of human capabilities and understanding.
Armed with a Master of Science degree and a Ph.D. in his field, Dr. Lowemann has consistently been at the forefront of research and innovation, delving into ways to optimize human performance, cognition, and overall well-being. His work at the Institute revolves around a profound commitment to harnessing cutting-edge science and technology to help individuals lead more fulfilling and intelligent lives.
Dr. Lowemann’s influence extends to the educational platform BetterSmarter.me, where he shares his insights, findings, and personal development strategies with a broader audience. His ongoing mission is shaping the way we perceive and leverage the vast capacities of the human mind, offering invaluable contributions to society’s overall success and collective well-being.