Communication interference identification is critical in electronic countermeasures. However, existed methods based on deep learning, such as convolutional neural networks (CNNs) and transformer, seldom take both local characteristics and global feature information of the signal into account. Motivated by the local convolution property of CNNs and the attention mechanism of transformer, we designed a novel network that combines both architectures, which make better use of both local and global characteristics of the signals. Additionally, recognizing the challenge of distinguishing contextual semantics within the one-dimensional signal data used in this study, we advocate the use of CNNs in place of word embedding, aligning more closely with the intrinsic features of the signal data. Furthermore, to capture the time-frequency characteristics of the signals, we integrate the proposed network with a cross-attention mechanism, facilitating the fusion of temporal and spectral domain feature information through multiple cross-attention computational layers. This innovation obviates the need for specialized time-frequency analysis. Experimental results demonstrate that our approach significantly improves recognition accuracy compared to existing methods, highlighting its efficacy in addressing the challenge of communication interference identification in electronic warfare.
Read Full Article (External Site)
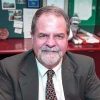
Dr. David Lowemann, M.Sc, Ph.D., is a co-founder of the Institute for the Future of Human Potential, where he leads the charge in pioneering Self-Enhancement Science for the Success of Society. With a keen interest in exploring the untapped potential of the human mind, Dr. Lowemann has dedicated his career to pushing the boundaries of human capabilities and understanding.
Armed with a Master of Science degree and a Ph.D. in his field, Dr. Lowemann has consistently been at the forefront of research and innovation, delving into ways to optimize human performance, cognition, and overall well-being. His work at the Institute revolves around a profound commitment to harnessing cutting-edge science and technology to help individuals lead more fulfilling and intelligent lives.
Dr. Lowemann’s influence extends to the educational platform BetterSmarter.me, where he shares his insights, findings, and personal development strategies with a broader audience. His ongoing mission is shaping the way we perceive and leverage the vast capacities of the human mind, offering invaluable contributions to society’s overall success and collective well-being.