Imagine you’re trying to find all the different types of birds in a forest. You come across a problem – some birds are so tiny that they get lost in the background! That’s exactly what happens with current semantic segmentation algorithms in computer vision. They struggle to capture small object information and suffer from feature distortion. It’s like looking for tiny birds without getting a clear view. But fear not, scientists have come up with a solution – CCSONet. This lightweight semantic segmentation network is like having a pair of binoculars that can zoom in on the tiniest birds while still maintaining lightning-fast speed. How does it work? Well, CCSONet has two powerful modules: LSCFEM and SOADM. The LSCFEM module allows for a broader and more flexible spatial range, eliminating feature distortion. On the other hand, the SOADM module enhances small object features by establishing correlations among objects of the same category. With this groundbreaking network, scientists achieved remarkable accuracy on Cityscapes and Camvid datasets without sacrificing speed! You can learn more about this exciting research by exploring the full article!
The current semantic segmentation algorithms suffer from encoding feature distortion and small object feature loss. Context information exchange can effectively address the feature distortion problem, but it has the issue of fixed spatial range. Maintaining the input feature resolution can reduce the loss of small object information but would slow down the network’s operation speed. To tackle these problems, we propose a lightweight semantic segmentation network with configurable context and small object attention (CCSONet). CCSONet includes a long-short distance configurable context feature enhancement module (LSCFEM) and a small object attention decoding module (SOADM). The LSCFEM differs from the regular context exchange module by configuring long and short-range relevant features for the current feature, providing a broader and more flexible spatial range. The SOADM enhances the features of small objects by establishing correlations among objects of the same category, avoiding the introduction of redundancy issues caused by high-resolution features. On the Cityscapes and Camvid datasets, our network achieves the accuracy of 76.9 mIoU and 73.1 mIoU, respectively, while maintaining speeds of 87 FPS and 138 FPS. It outperforms other lightweight semantic segmentation algorithms in terms of accuracy.
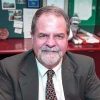
Dr. David Lowemann, M.Sc, Ph.D., is a co-founder of the Institute for the Future of Human Potential, where he leads the charge in pioneering Self-Enhancement Science for the Success of Society. With a keen interest in exploring the untapped potential of the human mind, Dr. Lowemann has dedicated his career to pushing the boundaries of human capabilities and understanding.
Armed with a Master of Science degree and a Ph.D. in his field, Dr. Lowemann has consistently been at the forefront of research and innovation, delving into ways to optimize human performance, cognition, and overall well-being. His work at the Institute revolves around a profound commitment to harnessing cutting-edge science and technology to help individuals lead more fulfilling and intelligent lives.
Dr. Lowemann’s influence extends to the educational platform BetterSmarter.me, where he shares his insights, findings, and personal development strategies with a broader audience. His ongoing mission is shaping the way we perceive and leverage the vast capacities of the human mind, offering invaluable contributions to society’s overall success and collective well-being.