Imagine decision-making as a complex dance, with biases as the music that guides our every move. To understand these biases, scientists have developed various models, each offering a unique perspective. Statistical models capture the data, but verbal theories often leave room for interpretation. Mathematical cognitive models, on the other hand, provide a structured framework with clear assumptions, enhancing prediction and simulation capabilities. By comparing signal detection theory to evidence accumulation models, researchers have found that the latter provide a more comprehensive understanding of decision-making by incorporating response time behavior. Exciting new studies explore attention and expectation biases using these evidence accumulation models, challenging previous beliefs and shedding light on their complex nature. Findings suggest that attention not only impacts the speed of evidence accumulation but also affects non-decision time. Similarly, prior probability influences both starting point and drift rate. These discoveries open doors to further investigation and fuel our curiosity to delve deeper into the fascinating world of decision-making biases.
Biases are a fundamental aspect of everyday life decision-making. A variety of modelling approaches have been suggested to capture decision-making biases. Statistical models are a means to describe the data, but the results are usually interpreted according to a verbal theory. This can lead to an ambiguous interpretation of the data. Mathematical cognitive models of decision-making outline the structure of the decision process with formal assumptions, providing advantages in terms of prediction, simulation, and interpretability compared to statistical models. We compare studies that used both signal detection theory and evidence accumulation models as models of decision-making biases, concluding that the latter provides a more comprehensive account of the decision-making phenomena by including response time behavior. We conclude by reviewing recent studies investigating attention and expectation biases with evidence accumulation models. Previous findings, reporting an exclusive influence of attention on the speed of evidence accumulation and prior probability on starting point, are challenged by novel results suggesting an additional effect of attention on non-decision time and prior probability on drift rate.
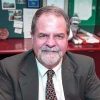
Dr. David Lowemann, M.Sc, Ph.D., is a co-founder of the Institute for the Future of Human Potential, where he leads the charge in pioneering Self-Enhancement Science for the Success of Society. With a keen interest in exploring the untapped potential of the human mind, Dr. Lowemann has dedicated his career to pushing the boundaries of human capabilities and understanding.
Armed with a Master of Science degree and a Ph.D. in his field, Dr. Lowemann has consistently been at the forefront of research and innovation, delving into ways to optimize human performance, cognition, and overall well-being. His work at the Institute revolves around a profound commitment to harnessing cutting-edge science and technology to help individuals lead more fulfilling and intelligent lives.
Dr. Lowemann’s influence extends to the educational platform BetterSmarter.me, where he shares his insights, findings, and personal development strategies with a broader audience. His ongoing mission is shaping the way we perceive and leverage the vast capacities of the human mind, offering invaluable contributions to society’s overall success and collective well-being.