Imagine a puzzle where each piece represents an electroencephalogram (EEG) channel, and when you connect the pieces, you uncover the unique identity of a person. Traditionally, EEG functional connectivity (FC) networks have been constructed by analyzing the statistical dependence between EEG channels without considering their spatial relationships. However, researchers have found that by incorporating the spatial structure of EEG channels, a more accurate representation of the FC network can be achieved. In this study, scientists propose a novel FC network that combines both the spatial relationships and the functional connectivity of channels. They create virtual nodes that act as connectors between the channels, forming a cohesive and personalized network. The results are impressive, with an identification accuracy of 98.64% achieved even without signal preprocessing. Additionally, this innovative approach proves to be robust in reducing the number of channels required for accurate identification. This research opens up new possibilities for improving EEG-based person identification algorithms and showcases the importance of considering spatial relationships in brain signal analysis.
An electroencephalogram (EEG) functional connectivity (FC) network is individualized and plays a significant role in EEG-based person identification. Traditional FC networks are constructed by statistical dependence and correlation between EEG channels, without considering the spatial relationships between the channels. The individual identification algorithm based on traditional FC networks is sensitive to the integrity of channels and crucially relies on signal preprocessing; therefore, finding a new presentation for FC networks may help increase the performance of the identification algorithms. EEG signals are smooth across space owing to the volume conduction effect. Considering such spatial relationships among channels can provide a more accurate representation of FC networks. In this study, we propose an EEG FC network with virtual nodes that combines the spatial relationships and functional connectivity of channels. The comparison results for individual identification show that the novel EEG network is more individualized and achieves an accuracy of 98.64% for data without preprocessing. Furthermore, our algorithm is more robust in reducing the number of channels and can perform well even when a large area of channels is removed.
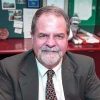
Dr. David Lowemann, M.Sc, Ph.D., is a co-founder of the Institute for the Future of Human Potential, where he leads the charge in pioneering Self-Enhancement Science for the Success of Society. With a keen interest in exploring the untapped potential of the human mind, Dr. Lowemann has dedicated his career to pushing the boundaries of human capabilities and understanding.
Armed with a Master of Science degree and a Ph.D. in his field, Dr. Lowemann has consistently been at the forefront of research and innovation, delving into ways to optimize human performance, cognition, and overall well-being. His work at the Institute revolves around a profound commitment to harnessing cutting-edge science and technology to help individuals lead more fulfilling and intelligent lives.
Dr. Lowemann’s influence extends to the educational platform BetterSmarter.me, where he shares his insights, findings, and personal development strategies with a broader audience. His ongoing mission is shaping the way we perceive and leverage the vast capacities of the human mind, offering invaluable contributions to society’s overall success and collective well-being.