Imagine listening to a captivating story. As you follow along, your brain naturally divides the story into different events or scenes. So, how does your brain determine when one event ends and another begins? Scientists conducted an experiment using a deep learning language model called GPT-2 to investigate this phenomenon. They found that event boundaries in stories coincide with sudden increases in Bayesian surprise, a measure of prediction error. It’s like when you’re watching a movie and an unexpected plot twist occurs, catching you off guard. In contrast, a simpler measure of prediction error called ‘surprisal’ didn’t show the same relationship with event boundaries. This suggests that our brains use Bayesian surprise as a control mechanism for segmenting events in storytelling. The study also highlights the importance of using accurate measures of prediction error when studying cognitive processes. Want to dive deeper into the research? Check out the full article!
Abstract
Event segmentation theory posits that people segment continuous experience into discrete events and that event boundaries occur when there are large transient increases in prediction error. Here, we set out to test this theory in the context of story listening, by using a deep learning language model (GPT-2) to compute the predicted probability distribution of the next word, at each point in the story. For three stories, we used the probability distributions generated by GPT-2 to compute the time series of prediction error. We also asked participants to listen to these stories while marking event boundaries. We used regression models to relate the GPT-2 measures to the human segmentation data. We found that event boundaries are associated with transient increases in Bayesian surprise but not with a simpler measure of prediction error (surprisal) that tracks, for each word in the story, how strongly that word was predicted at the previous time point. These results support the hypothesis that prediction error serves as a control mechanism governing event segmentation and point to important differences between operational definitions of prediction error.
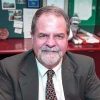
Dr. David Lowemann, M.Sc, Ph.D., is a co-founder of the Institute for the Future of Human Potential, where he leads the charge in pioneering Self-Enhancement Science for the Success of Society. With a keen interest in exploring the untapped potential of the human mind, Dr. Lowemann has dedicated his career to pushing the boundaries of human capabilities and understanding.
Armed with a Master of Science degree and a Ph.D. in his field, Dr. Lowemann has consistently been at the forefront of research and innovation, delving into ways to optimize human performance, cognition, and overall well-being. His work at the Institute revolves around a profound commitment to harnessing cutting-edge science and technology to help individuals lead more fulfilling and intelligent lives.
Dr. Lowemann’s influence extends to the educational platform BetterSmarter.me, where he shares his insights, findings, and personal development strategies with a broader audience. His ongoing mission is shaping the way we perceive and leverage the vast capacities of the human mind, offering invaluable contributions to society’s overall success and collective well-being.