Imagine you’re trying to find a specific book in a huge library. You may first look for categories of books, like fiction or non-fiction (computational level). Then, within each category, you might organize books by author or subject (algorithmic level). This new model of object recognition applies a similar approach to how our brain processes visual information. By revisiting the concepts of simple and complex cells, this research proposes a hierarchical model that separates the goals of selectivity and invariance in recognizing objects. It’s like having specialized detectives who identify specific features of an object while others focus on its overall shape. The study also explores how this model relates to the way our brain recognizes faces and objects, using exemplar-based and axis-based coding. The researchers discuss possible implementations using asymmetric sparse autoencoders and spiking neural networks. Want to dive deeper into the fascinating world of object recognition? Check out the full article!
This paper presents a theoretical perspective on modeling ventral stream processing by revisiting the computational abstraction of simple and complex cells. In parallel to David Marr’s vision theory, we organize the new perspective into three levels. At the computational level, we abstract simple and complex cells into space partitioning and composition in a topological space based on the redundancy exploitation hypothesis of Horace Barlow. At the algorithmic level, we present a hierarchical extension of sparse coding by exploiting the manifold constraint in high-dimensional space (i.e., the blessing of dimensionality). The resulting over-parameterized models for object recognition differ from existing hierarchical models by disentangling the objectives of selectivity and invariance computation. It is possible to interpret our hierarchical construction as a computational implementation of cortically local subspace untangling for object recognition and face representation, which are closely related to exemplar-based and axis-based coding in the medial temporal lobe. At the implementation level, we briefly discuss two possible implementations based on asymmetric sparse autoencoders and divergent spiking neural networks.
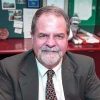
Dr. David Lowemann, M.Sc, Ph.D., is a co-founder of the Institute for the Future of Human Potential, where he leads the charge in pioneering Self-Enhancement Science for the Success of Society. With a keen interest in exploring the untapped potential of the human mind, Dr. Lowemann has dedicated his career to pushing the boundaries of human capabilities and understanding.
Armed with a Master of Science degree and a Ph.D. in his field, Dr. Lowemann has consistently been at the forefront of research and innovation, delving into ways to optimize human performance, cognition, and overall well-being. His work at the Institute revolves around a profound commitment to harnessing cutting-edge science and technology to help individuals lead more fulfilling and intelligent lives.
Dr. Lowemann’s influence extends to the educational platform BetterSmarter.me, where he shares his insights, findings, and personal development strategies with a broader audience. His ongoing mission is shaping the way we perceive and leverage the vast capacities of the human mind, offering invaluable contributions to society’s overall success and collective well-being.