Just as a skilled detective uses various clues to solve a mystifying case, scientists have developed a complexity-based graph convolutional neural network (GCNN) that can decipher the different stages of epilepsy. Imagine looking at a graph with multiple interconnected points representing brain activity during epileptogenesis. This groundbreaking GCNN analyzes five complexity indicators, such as approximate entropy and Kolmogorov complexity, to accurately identify the normal, acute, and chronic phases of epilepsy. By feeding multi-channel EEG complexity data into the GCNN model, it achieves an impressive prediction accuracy of over 98% and an F1 score exceeding 97% for each individual rat. These findings reinforce the significance of EEG complexity characteristics in accurately recognizing different stages of epilepsy. To delve deeper into this fascinating research and its implications for epilepsy diagnosis and treatment, check out the full article!
IntroductionThe automatic precision detection technology based on electroencephalography (EEG) is essential in epilepsy studies. It can provide objective proof for epilepsy diagnosis, treatment, and evaluation, thus helping doctors improve treatment efficiency. At present, the normal and acute phases of epilepsy can be well identified through EEG analysis, but distinguishing between the normal and chronic phases is still tricky.MethodsIn this paper, five popular complexity indicators of EEG signal, including approximate entropy, sample entropy, permutation entropy, fuzzy entropy and Kolmogorov complexity, are computed from rat hippocampi to characterize the normal, acute, and chronic phases during epileptogenesis. Results of one-way ANOVA and principal component analysis both show that utilizing complexity features, we are able to easily identify differences between normal, acute, and chronic phases. We also propose an innovative framework for epilepsy detection based on graph convolutional neural network (GCNN) using multi-channel EEG complexity as input.ResultsCombining information of five complexity measures at eight channels, our GCNN model demonstrate superior ability in recognizing the normal, acute, and chronic phases. Experiments results show that our GCNN model reached the high prediction accuracy above 98% and F1 score above 97% among these three phases for each individual rat.DiscussionOur research practice based on real data shows that EEG complexity characteristics are of great significance for recognizing different stages of epilepsy.
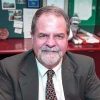
Dr. David Lowemann, M.Sc, Ph.D., is a co-founder of the Institute for the Future of Human Potential, where he leads the charge in pioneering Self-Enhancement Science for the Success of Society. With a keen interest in exploring the untapped potential of the human mind, Dr. Lowemann has dedicated his career to pushing the boundaries of human capabilities and understanding.
Armed with a Master of Science degree and a Ph.D. in his field, Dr. Lowemann has consistently been at the forefront of research and innovation, delving into ways to optimize human performance, cognition, and overall well-being. His work at the Institute revolves around a profound commitment to harnessing cutting-edge science and technology to help individuals lead more fulfilling and intelligent lives.
Dr. Lowemann’s influence extends to the educational platform BetterSmarter.me, where he shares his insights, findings, and personal development strategies with a broader audience. His ongoing mission is shaping the way we perceive and leverage the vast capacities of the human mind, offering invaluable contributions to society’s overall success and collective well-being.