Just like how some people are really good at specific tasks but struggle with general ones, researchers in artificial intelligence have been exploring two different approaches to understanding human reasoning: task-specific models and domain-general mapping. In this study, scientists focused on visual analogical reasoning using images of three-dimensional objects. They compared human performance to that of two deep learning models, which were specifically trained on these analogy problems, and a new model they developed called the part-based comparison (PCM) model. The results showed that the domain-general PCM model performed similarly to humans in key aspects of analogical reasoning, while the task-specific deep learning models fell short. This suggests that achieving human-like analogical reasoning may not be solely achieved through training specific models on large datasets for a particular type of analogy. Instead, it seems that humans (and potentially machines) achieve analogical reasoning by learning representations that encode structural information useful for multiple tasks and by efficiently computing relational similarity. To learn more about this fascinating research, check out the full article!
Abstract
Advances in artificial intelligence have raised a basic question about human intelligence: Is human reasoning best emulated by applying task-specific knowledge acquired from a wealth of prior experience, or is it based on the domain-general manipulation and comparison of mental representations? We address this question for the case of visual analogical reasoning. Using realistic images of familiar three-dimensional objects (cars and their parts), we systematically manipulated viewpoints, part relations, and entity properties in visual analogy problems. We compared human performance to that of two recent deep learning models (Siamese Network and Relation Network) that were directly trained to solve these problems and to apply their task-specific knowledge to analogical reasoning. We also developed a new model using part-based comparison (PCM) by applying a domain-general mapping procedure to learned representations of cars and their component parts. Across four-term analogies (Experiment 1) and open-ended analogies (Experiment 2), the domain-general PCM model, but not the task-specific deep learning models, generated performance similar in key aspects to that of human reasoners. These findings provide evidence that human-like analogical reasoning is unlikely to be achieved by applying deep learning with big data to a specific type of analogy problem. Rather, humans do (and machines might) achieve analogical reasoning by learning representations that encode structural information useful for multiple tasks, coupled with efficient computation of relational similarity.
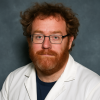
Dr. David Lowemann, M.Sc, Ph.D., is a co-founder of the Institute for the Future of Human Potential, where he leads the charge in pioneering Self-Enhancement Science for the Success of Society. With a keen interest in exploring the untapped potential of the human mind, Dr. Lowemann has dedicated his career to pushing the boundaries of human capabilities and understanding.
Armed with a Master of Science degree and a Ph.D. in his field, Dr. Lowemann has consistently been at the forefront of research and innovation, delving into ways to optimize human performance, cognition, and overall well-being. His work at the Institute revolves around a profound commitment to harnessing cutting-edge science and technology to help individuals lead more fulfilling and intelligent lives.
Dr. Lowemann’s influence extends to the educational platform BetterSmarter.me, where he shares his insights, findings, and personal development strategies with a broader audience. His ongoing mission is shaping the way we perceive and leverage the vast capacities of the human mind, offering invaluable contributions to society’s overall success and collective well-being.