Imagine you’re trying to solve a complex puzzle, but you have no idea how you actually did it. That’s how scientists feel when they use deep learning models for EEG-based brain computer interfaces (BCI). These models have achieved remarkable performance, but researchers are still scratching their heads about how they work. To shed some light on this mystery, scientists have been developing interpretation techniques that generate heatmaps to show which parts of the input are most important for the model’s decision-making. But here’s the twist: it turns out that these interpretation results can’t always be trusted! The accuracy and reliability of these heatmaps vary depending on factors like the interpretation technique used, model structure, and dataset types. So, scientists conducted studies to evaluate seven different interpretation techniques across various models and datasets. Their findings highlight the need to carefully select an appropriate interpretation technique and caution against blindly relying on interpretation results. Based on their observations, they propose a set of procedures to ensure the interpretation results are understandable and trustworthy. This research opens up new possibilities for improving EEG-based BCIs by better understanding and interpreting deep learning models.
IntroductionAs deep learning has achieved state-of-the-art performance for many tasks of EEG-based BCI, many efforts have been made in recent years trying to understand what have been learned by the models. This is commonly done by generating a heatmap indicating to which extent each pixel of the input contributes to the final classification for a trained model. Despite the wide use, it is not yet understood to which extent the obtained interpretation results can be trusted and how accurate they can reflect the model decisions.MethodsWe conduct studies to quantitatively evaluate seven different deep interpretation techniques across different models and datasets for EEG-based BCI.ResultsThe results reveal the importance of selecting a proper interpretation technique as the initial step. In addition, we also find that the quality of the interpretation results is inconsistent for individual samples despite when a method with an overall good performance is used. Many factors, including model structure and dataset types, could potentially affect the quality of the interpretation results.DiscussionBased on the observations, we propose a set of procedures that allow the interpretation results to be presented in an understandable and trusted way. We illustrate the usefulness of our method for EEG-based BCI with instances selected from different scenarios.
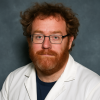
Dr. David Lowemann, M.Sc, Ph.D., is a co-founder of the Institute for the Future of Human Potential, where he leads the charge in pioneering Self-Enhancement Science for the Success of Society. With a keen interest in exploring the untapped potential of the human mind, Dr. Lowemann has dedicated his career to pushing the boundaries of human capabilities and understanding.
Armed with a Master of Science degree and a Ph.D. in his field, Dr. Lowemann has consistently been at the forefront of research and innovation, delving into ways to optimize human performance, cognition, and overall well-being. His work at the Institute revolves around a profound commitment to harnessing cutting-edge science and technology to help individuals lead more fulfilling and intelligent lives.
Dr. Lowemann’s influence extends to the educational platform BetterSmarter.me, where he shares his insights, findings, and personal development strategies with a broader audience. His ongoing mission is shaping the way we perceive and leverage the vast capacities of the human mind, offering invaluable contributions to society’s overall success and collective well-being.