The brain is like a complex puzzle, and understanding its signals is key to diagnosing and treating epilepsy. In a recent study, scientists developed a cutting-edge technique called Deep Extreme Learning Machine (DELM) to analyze electroencephalogram (EEG) signals and accurately predict epileptic seizures. Inspired by the stacking generalization principle, DELM is a hierarchical network made up of multiple independent ELM modules. Think of it like a team of expert detectives working together to solve a case. One unique feature of DELM is its ability to incorporate augmented knowledge from the EEG signals, enhancing the overall understanding of the brain’s activity. This process is quick and efficient, avoiding the costly fine-tuning of parameters that other approaches require. The results of extensive experiments on real EEG data showed that DELM outperformed traditional methods and achieved impressive accuracy in predicting seizures. Not only that, but DELM also proved to be more computationally efficient, enabling faster real-time detection of seizure onset. This research holds great promise for improving epilepsy diagnosis and advancing our understanding of the brain’s intricate signals.
IntroductionIntelligent recognition of electroencephalogram (EEG) signals can remarkably improve the accuracy of epileptic seizure prediction, which is essential for epileptic diagnosis. Extreme learning machine (ELM) has been applied to EEG signals recognition, however, the artifacts and noises in EEG signals have a serious effect on recognition efficiency. Deep learning is capable of noise resistance, contributing to removing the noise in raw EEG signals. But traditional deep networks suffer from time-consuming training and slow convergence.MethodsTherefore, a novel deep learning based ELM (denoted as DELM) motivated by stacking generalization principle is proposed in this paper. Deep extreme learning machine (DELM) is a hierarchical network composed of several independent ELM modules. Augmented EEG knowledge is taken as complementary component, which will then be mapped into next module. This learning process is so simple and fast, meanwhile, it can excavate the implicit knowledge in raw data to a greater extent. Additionally, the proposed method is operated in a single-direction manner, so there is no need to perform parameters fine-tuning, which saves the expense of time.ResultsExtensive experiments are conducted on the public Bonn EEG dataset. The experimental results demonstrate that compared with the commonly-used seizure prediction methods, the proposed DELM wins the best average accuracies in 13 out of the 22 data and the best average F-measure scores in 10 out of the 22 data. And the running time of DELM is more than two times quickly than deep learning methods.DiscussionTherefore, DELM is superior to traditional and some state-of-the-art machine learning methods. The proposed architecture demonstrates its feasibility and superiority in epileptic EEG signal recognition. The proposed less computationally intensive deep classifier enables faster seizure onset detection, which is showing great potential on the application of real-time EEG signal classification.
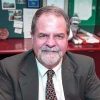
Dr. David Lowemann, M.Sc, Ph.D., is a co-founder of the Institute for the Future of Human Potential, where he leads the charge in pioneering Self-Enhancement Science for the Success of Society. With a keen interest in exploring the untapped potential of the human mind, Dr. Lowemann has dedicated his career to pushing the boundaries of human capabilities and understanding.
Armed with a Master of Science degree and a Ph.D. in his field, Dr. Lowemann has consistently been at the forefront of research and innovation, delving into ways to optimize human performance, cognition, and overall well-being. His work at the Institute revolves around a profound commitment to harnessing cutting-edge science and technology to help individuals lead more fulfilling and intelligent lives.
Dr. Lowemann’s influence extends to the educational platform BetterSmarter.me, where he shares his insights, findings, and personal development strategies with a broader audience. His ongoing mission is shaping the way we perceive and leverage the vast capacities of the human mind, offering invaluable contributions to society’s overall success and collective well-being.