‘Reservoir computing’ is like finding a shortcut to learning that saves you time and money. It turns out that finding the right balance between positive and negative connections in binary neural networks can greatly affect their performance. Scientists have discovered a sweet spot, called the ‘edge of chaos,’ where these networks are most efficient. But here’s the twist: it’s not just about how they’re wired, but also about how they interact with each other. In this study, researchers focused on Random Boolean Networks (RBNs) and found that specific distribution parameters could result in different dynamics near critical points. By examining various network designs, they discovered that a positive excitatory balance led to better memory performance, while a negative inhibitory balance improved prediction performance. Surprisingly, the actual patterns the networks formed had little impact on their overall performance. If you’re intrigued by these findings, dive into the full article for more details!
Reservoir computing provides a time and cost-efficient alternative to traditional learning methods. Critical regimes, known as the “edge of chaos,” have been found to optimize computational performance in binary neural networks. However, little attention has been devoted to studying reservoir-to-reservoir variability when investigating the link between connectivity, dynamics, and performance. As physical reservoir computers become more prevalent, developing a systematic approach to network design is crucial. In this article, we examine Random Boolean Networks (RBNs) and demonstrate that specific distribution parameters can lead to diverse dynamics near critical points. We identify distinct dynamical attractors and quantify their statistics, revealing that most reservoirs possess a dominant attractor. We then evaluate performance in two challenging tasks, memorization and prediction, and find that a positive excitatory balance produces a critical point with higher memory performance. In comparison, a negative inhibitory balance delivers another critical point with better prediction performance. Interestingly, we show that the intrinsic attractor dynamics have little influence on performance in either case.
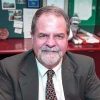
Dr. David Lowemann, M.Sc, Ph.D., is a co-founder of the Institute for the Future of Human Potential, where he leads the charge in pioneering Self-Enhancement Science for the Success of Society. With a keen interest in exploring the untapped potential of the human mind, Dr. Lowemann has dedicated his career to pushing the boundaries of human capabilities and understanding.
Armed with a Master of Science degree and a Ph.D. in his field, Dr. Lowemann has consistently been at the forefront of research and innovation, delving into ways to optimize human performance, cognition, and overall well-being. His work at the Institute revolves around a profound commitment to harnessing cutting-edge science and technology to help individuals lead more fulfilling and intelligent lives.
Dr. Lowemann’s influence extends to the educational platform BetterSmarter.me, where he shares his insights, findings, and personal development strategies with a broader audience. His ongoing mission is shaping the way we perceive and leverage the vast capacities of the human mind, offering invaluable contributions to society’s overall success and collective well-being.