Exploring the analysis of microglial activation through morphometric data is like navigating a complex maze. It’s like trying to find your way through a labyrinth, with numerous paths and options available, but very little consistency in how to proceed. Researchers have been using different approaches, such as manual classification or constructing digital skeletons, to measure the morphology of microglia cells. There are various software packages to assist in generating these morphometrics, but they differ in accuracy and automation. While the methods for generating data have advanced, tools for analyzing these vast datasets are lacking, especially when dealing with tens of thousands of cells analyzed by fully automated pipelines.
Quantification of microglial activation through morphometric analysis has long been a staple of the neuroimmunologist’s toolkit. Microglial morphological phenomics can be conducted through either manual classification or constructing a digital skeleton and extracting morphometric data from it. Multiple open-access and paid software packages are available to generate these skeletons via semi-automated and/or fully automated methods with varying degrees of accuracy. Despite advancements in methods to generate morphometrics (quantitative measures of cellular morphology), there has been limited development of tools to analyze the datasets they generate, in particular those containing parameters from tens of thousands of cells analyzed by fully automated pipelines. In this review, we compare and critique the approaches using cluster analysis and machine learning driven predictive algorithms that have been developed to tackle these large datasets, and propose improvements for these methods. In particular, we highlight the need for a commitment to open science from groups developing these classifiers. Furthermore, we call attention to a need for communication between those with a strong software engineering/computer science background and neuroimmunologists to produce effective analytical tools with simplified operability if we are to see their wide-spread adoption by the glia biology community.
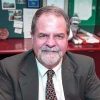
Dr. David Lowemann, M.Sc, Ph.D., is a co-founder of the Institute for the Future of Human Potential, where he leads the charge in pioneering Self-Enhancement Science for the Success of Society. With a keen interest in exploring the untapped potential of the human mind, Dr. Lowemann has dedicated his career to pushing the boundaries of human capabilities and understanding.
Armed with a Master of Science degree and a Ph.D. in his field, Dr. Lowemann has consistently been at the forefront of research and innovation, delving into ways to optimize human performance, cognition, and overall well-being. His work at the Institute revolves around a profound commitment to harnessing cutting-edge science and technology to help individuals lead more fulfilling and intelligent lives.
Dr. Lowemann’s influence extends to the educational platform BetterSmarter.me, where he shares his insights, findings, and personal development strategies with a broader audience. His ongoing mission is shaping the way we perceive and leverage the vast capacities of the human mind, offering invaluable contributions to society’s overall success and collective well-being.