Imagine being able to detect Alzheimer’s disease just by listening to someone speak. Researchers have reviewed the potential of using spontaneous speech as a non-invasive and cost-effective method for detecting this debilitating condition. In Alzheimer’s patients, language skills deteriorate, making speech an insightful tool for diagnosis. This review paper dives into the technologies used for automatic detection, including data collection, feature extraction, and classification. Think of it like analyzing the different notes and tones in someone’s voice to unravel the signs of Alzheimer’s. The paper explores different techniques such as extracting acoustic features from speech and linguistic features from text. It also touches on optimization strategies to improve AD detection systems. However, challenges like managing large data sets and integrating multiple sources of information remain. Still, there is so much potential for further research in this fascinating field! If you’re curious about the science behind detecting Alzheimer’s through speech, be sure to check out the full article.
Alzheimer’s disease (AD) is considered as one of the leading causes of death among people over the age of 70 that is characterized by memory degradation and language impairment. Due to language dysfunction observed in individuals with AD patients, the speech-based methods offer non-invasive, convenient, and cost-effective solutions for the automatic detection of AD. This paper systematically reviews the technologies to detect the onset of AD from spontaneous speech, including data collection, feature extraction and classification. First the paper formulates the task of automatic detection of AD and describes the process of data collection. Then, feature extractors from speech data and transcripts are reviewed, which mainly contains acoustic features from speech and linguistic features from text. Especially, general handcrafted features and deep embedding features are organized from different modalities. Additionally, this paper summarizes optimization strategies for AD detection systems. Finally, the paper addresses challenges related to data size, model explainability, reliability and multimodality fusion, and discusses potential research directions based on these challenges.
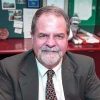
Dr. David Lowemann, M.Sc, Ph.D., is a co-founder of the Institute for the Future of Human Potential, where he leads the charge in pioneering Self-Enhancement Science for the Success of Society. With a keen interest in exploring the untapped potential of the human mind, Dr. Lowemann has dedicated his career to pushing the boundaries of human capabilities and understanding.
Armed with a Master of Science degree and a Ph.D. in his field, Dr. Lowemann has consistently been at the forefront of research and innovation, delving into ways to optimize human performance, cognition, and overall well-being. His work at the Institute revolves around a profound commitment to harnessing cutting-edge science and technology to help individuals lead more fulfilling and intelligent lives.
Dr. Lowemann’s influence extends to the educational platform BetterSmarter.me, where he shares his insights, findings, and personal development strategies with a broader audience. His ongoing mission is shaping the way we perceive and leverage the vast capacities of the human mind, offering invaluable contributions to society’s overall success and collective well-being.