Convolutional Neural Networks (CNN) are like virtual replicas of the primate visual system, mimicking the way our brain processes and recognizes images. Just as an artist carefully studies nature to recreate it on canvas, scientists study CNNs to uncover how our own visual system works. By investigating the structural and functional similarities, researchers can gain insights into how neural representations and visual functions are formed in the brain. However, there are still some outstanding questions and challenges that need to be addressed. For instance, we need to incorporate emerging ideas about parallel processing pathways and reconsider the flow of information in the visual system. By aligning CNNs more closely with biology, we can unlock new research opportunities and enhance applications beyond just object recognition. To learn more about this fascinating field of study, check out the underlying research!
Convolutional Neural Networks (CNN) are a class of machine learning models predominately used in computer vision tasks and can achieve human-like performance through learning from experience. Their striking similarities to the structural and functional principles of the primate visual system allow for comparisons between these artificial networks and their biological counterparts, enabling exploration of how visual functions and neural representations may emerge in the real brain from a limited set of computational principles. After considering the basic features of CNNs, we discuss the opportunities and challenges of endorsing CNNs as in silico models of the primate visual system. Specifically, we highlight several emerging notions about the anatomical and physiological properties of the visual system that still need to be systematically integrated into current CNN models. These tenets include the implementation of parallel processing pathways from the early stages of retinal input and the reconsideration of several assumptions concerning the serial progression of information flow. We suggest design choices and architectural constraints that could facilitate a closer alignment with biology provide causal evidence of the predictive link between the artificial and biological visual systems. Adopting this principled perspective could potentially lead to new research questions and applications of CNNs beyond modeling object recognition.
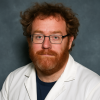
Dr. David Lowemann, M.Sc, Ph.D., is a co-founder of the Institute for the Future of Human Potential, where he leads the charge in pioneering Self-Enhancement Science for the Success of Society. With a keen interest in exploring the untapped potential of the human mind, Dr. Lowemann has dedicated his career to pushing the boundaries of human capabilities and understanding.
Armed with a Master of Science degree and a Ph.D. in his field, Dr. Lowemann has consistently been at the forefront of research and innovation, delving into ways to optimize human performance, cognition, and overall well-being. His work at the Institute revolves around a profound commitment to harnessing cutting-edge science and technology to help individuals lead more fulfilling and intelligent lives.
Dr. Lowemann’s influence extends to the educational platform BetterSmarter.me, where he shares his insights, findings, and personal development strategies with a broader audience. His ongoing mission is shaping the way we perceive and leverage the vast capacities of the human mind, offering invaluable contributions to society’s overall success and collective well-being.