Imagine you have a simulator that mimics the growth, migration, and pruning of the brain. That’s exactly what NeuroDevSim does! It’s like having your own little brain laboratory, where you can watch as neurons extend, branch, and terminate their growth. You can even witness somatic fronts migrating to new positions and parts of neurons being pruned. But how does it work? Well, NeuroDevSim uses an agent-based modeling approach to simulate these processes. Each cycle, different agents called fronts carry out specific tasks, like extending dendrites or axons, or pruning neuron parts. The best part is, NeuroDevSim is not only efficient, but it also utilizes shared memory parallelization to achieve incredible computational speed without the need for messaging between cores. With parallel processing on up to 96 cores for large models, it’s like having an army of researchers all working together! So if you’re interested in the fascinating world of neural development and want to explore NeuroDevSim further, check out the code on GitHub and get ready for some mind-blowing simulations!
The Neural Development Simulator, NeuroDevSim, is a Python module that simulates the most important aspects of brain development: morphological growth, migration, and pruning. It uses an agent-based modeling approach inherited from the NeuroMaC software. Each cycle has agents called fronts execute model-specific code. In the case of a growing dendritic or axonal front, this will be a choice between extension, branching, or growth termination. Somatic fronts can migrate to new positions and any front can be retracted to prune parts of neurons. Collision detection prevents new or migrating fronts from overlapping with existing ones. NeuroDevSim is a multi-core program that uses an innovative shared memory approach to achieve parallel processing without messaging. We demonstrate linear strong parallel scaling up to 96 cores for large models and have run these successfully on 128 cores. Most of the shared memory parallelism is achieved without memory locking. Instead, cores have only write privileges to private sections of arrays, while being able to read the entire shared array. Memory conflicts are avoided by a coding rule that allows only active fronts to use methods that need writing access. The exception is collision detection, which is needed to avoid the growth of physically overlapping structures. For collision detection, a memory-locking mechanism was necessary to control access to grid points that register the location of nearby fronts. A custom approach using a serialized lock broker was able to manage both read and write locking. NeuroDevSim allows easy modeling of most aspects of neural development for models simulating a few complex or thousands of simple neurons or a mixture of both.Code available athttps://github.com/CNS-OIST/NeuroDevSim.
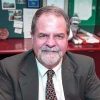
Dr. David Lowemann, M.Sc, Ph.D., is a co-founder of the Institute for the Future of Human Potential, where he leads the charge in pioneering Self-Enhancement Science for the Success of Society. With a keen interest in exploring the untapped potential of the human mind, Dr. Lowemann has dedicated his career to pushing the boundaries of human capabilities and understanding.
Armed with a Master of Science degree and a Ph.D. in his field, Dr. Lowemann has consistently been at the forefront of research and innovation, delving into ways to optimize human performance, cognition, and overall well-being. His work at the Institute revolves around a profound commitment to harnessing cutting-edge science and technology to help individuals lead more fulfilling and intelligent lives.
Dr. Lowemann’s influence extends to the educational platform BetterSmarter.me, where he shares his insights, findings, and personal development strategies with a broader audience. His ongoing mission is shaping the way we perceive and leverage the vast capacities of the human mind, offering invaluable contributions to society’s overall success and collective well-being.