Imagine building a puzzle that requires you to combine puzzle pieces from multiple sets. In the quest to better understand Alzheimer’s disease (AD), scientists have developed a joint genetic score called MetaGRS. They integrated genetic information from AD and 24 other traits, analyzing data from two independent cohorts. When compared to the traditional AD genetic risk score (GRS), MetaGRS showed a striking similarity in its ability to predict AD risk. A one standard deviation increase in MetaGRS resulted in a 57% increase in AD risk, just slightly less than the increase observed with the AD GRS alone. Furthermore, when they examined the impact of a specific gene variant called APOE e4, they found that MetaGRS did not provide a substantial improvement over the traditional model. However, the MetaGRS still outperformed a predictive model without any genetic information. This research showcases the potential of multi-trait genetic modeling to enhance our ability to predict Alzheimer’s disease. Want to dig deeper into the study? Check out the full article!
To better capture the polygenic architecture of Alzheimer’s disease (AD), we developed a joint genetic score, MetaGRS. We incorporated genetic variants for AD and 24 other traits from two independent cohorts, NACC (n = 3,174, training set) and UPitt (n = 2,053, validation set). One standard deviation increase in the MetaGRS is associated with about 57% increase in the AD risk [hazard ratio (HR) = 1.577, p = 7.17 E-56], showing little difference from the HR for AD GRS alone (HR = 1.579, p = 1.20E-56), suggesting similar utility of both models. We also conducted APOE-stratified analyses to assess the role of the e4 allele on risk prediction. Similar to that of the combined model, our stratified results did not show a considerable improvement of the MetaGRS. Our study showed that the prediction power of the MetaGRS significantly outperformed that of the reference model without any genetic information, but was effectively equivalent to the prediction power of the AD GRS.
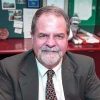
Dr. David Lowemann, M.Sc, Ph.D., is a co-founder of the Institute for the Future of Human Potential, where he leads the charge in pioneering Self-Enhancement Science for the Success of Society. With a keen interest in exploring the untapped potential of the human mind, Dr. Lowemann has dedicated his career to pushing the boundaries of human capabilities and understanding.
Armed with a Master of Science degree and a Ph.D. in his field, Dr. Lowemann has consistently been at the forefront of research and innovation, delving into ways to optimize human performance, cognition, and overall well-being. His work at the Institute revolves around a profound commitment to harnessing cutting-edge science and technology to help individuals lead more fulfilling and intelligent lives.
Dr. Lowemann’s influence extends to the educational platform BetterSmarter.me, where he shares his insights, findings, and personal development strategies with a broader audience. His ongoing mission is shaping the way we perceive and leverage the vast capacities of the human mind, offering invaluable contributions to society’s overall success and collective well-being.