Imagine you’re trying to build a high-performance race car, but you want to streamline the process and get faster results. That’s where the Functional Extreme Learning Machine (FELM) comes in. Just like a skilled mechanic who knows all the ins and outs of building a powerful engine, the FELM uses functional neurons as its basic units, guided by the principles of functional equation solving theory. By adjusting the coefficients of the basis functions in these neurons, the FELM learns and adapts, making it a lightning-fast and precise learning algorithm. In fact, it outperforms traditional methods like the ELM and support vector machine (SVM) in achieving better performance on regression problems. With its simple yet powerful approach, the FELM shows great promise for revolutionizing the field of machine learning.
To dive deeper into the details of this groundbreaking research, check out the full article linked above!
IntroductionExtreme learning machine (ELM) is a training algorithm for single hidden layer feedforward neural network (SLFN), which converges much faster than traditional methods and yields promising performance. However, the ELM also has some shortcomings, such as structure selection, overfitting and low generalization performance.MethodsThis article a new functional neuron (FN) model is proposed, we takes functional neurons as the basic unit, and uses functional equation solving theory to guide the modeling process of FELM, a new functional extreme learning machine (FELM) model theory is proposed.ResultsThe FELM implements learning by adjusting the coefficients of the basis function in neurons. At the same time, a simple, iterative-free and high-precision fast parameter learning algorithm is proposed.DiscussionThe standard data sets UCI and StatLib are selected for regression problems, and compared with the ELM, support vector machine (SVM) and other algorithms, the experimental results show that the FELM achieves better performance.
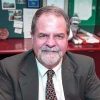
Dr. David Lowemann, M.Sc, Ph.D., is a co-founder of the Institute for the Future of Human Potential, where he leads the charge in pioneering Self-Enhancement Science for the Success of Society. With a keen interest in exploring the untapped potential of the human mind, Dr. Lowemann has dedicated his career to pushing the boundaries of human capabilities and understanding.
Armed with a Master of Science degree and a Ph.D. in his field, Dr. Lowemann has consistently been at the forefront of research and innovation, delving into ways to optimize human performance, cognition, and overall well-being. His work at the Institute revolves around a profound commitment to harnessing cutting-edge science and technology to help individuals lead more fulfilling and intelligent lives.
Dr. Lowemann’s influence extends to the educational platform BetterSmarter.me, where he shares his insights, findings, and personal development strategies with a broader audience. His ongoing mission is shaping the way we perceive and leverage the vast capacities of the human mind, offering invaluable contributions to society’s overall success and collective well-being.