Understanding the decisions made by deep neural networks is like examining the thought process behind a magic trick. In this study, researchers aimed to uncover the inner workings of these networks by investigating and interpreting their outputs. They focused on analyzing how perturbations in the training dataset affected the model’s predictions. By calculating influence scores at different layers of the network, they were able to identify influential training images that impacted the testing results. This allowed them to uncover biases and understand why certain predictions were made. The findings demonstrated that layer-wise influence analysis, combined with local interpretability methods, was effective in revealing significant differences in disturbed image subgroups. Overall, this research provides valuable insights into understanding and potentially retraining deep learning models to reduce biases and improve their interpretability.
An understanding of deep neural network decisions is based on the interpretability of model, which provides explanations that are understandable to human beings and helps avoid biases in model predictions. This study investigates and interprets the model output based on images from the training dataset, i.e., to debug the results of a network model in relation to the training dataset. Our objective was to understand the behavior (specifically, class prediction) of deep learning models through the analysis of perturbations of the loss functions. We calculated influence scores for the VGG16 network at different hidden layers across three types of disturbances in the original images of the ImageNet dataset: texture, style, and background elimination. The global and layer-wise influence scores allowed the identification of the most influential training images for the given testing set. We illustrated our findings using influence scores by highlighting the types of disturbances that bias predictions of the network. According to our results, layer-wise influence analysis pairs well with local interpretability methods such as Shapley values to demonstrate significant differences between disturbed image subgroups. Particularly in an image classification task, our layer-wise interpretability approach plays a pivotal role to identify the classification bias in pre-trained convolutional neural networks, thus, providing useful insights to retrain specific hidden layers.
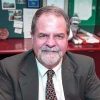
Dr. David Lowemann, M.Sc, Ph.D., is a co-founder of the Institute for the Future of Human Potential, where he leads the charge in pioneering Self-Enhancement Science for the Success of Society. With a keen interest in exploring the untapped potential of the human mind, Dr. Lowemann has dedicated his career to pushing the boundaries of human capabilities and understanding.
Armed with a Master of Science degree and a Ph.D. in his field, Dr. Lowemann has consistently been at the forefront of research and innovation, delving into ways to optimize human performance, cognition, and overall well-being. His work at the Institute revolves around a profound commitment to harnessing cutting-edge science and technology to help individuals lead more fulfilling and intelligent lives.
Dr. Lowemann’s influence extends to the educational platform BetterSmarter.me, where he shares his insights, findings, and personal development strategies with a broader audience. His ongoing mission is shaping the way we perceive and leverage the vast capacities of the human mind, offering invaluable contributions to society’s overall success and collective well-being.