Imagine you’re a scientist on a mission to uncover hidden treasures. You gather your trusty tools – Gradient boosting neural network, Deep neural network, and Deep Forest – and embark on an exciting adventure. In this study, researchers developed a groundbreaking method called EnGDD to predict drug-target interactions. Like an archaeologist sifting through layers of soil, EnGDD goes through feature acquisition, dimensional reduction, and DTI classification to uncover valuable information. And boy, did it deliver! Compared to other methods, EnGDD showed superior performance in identifying potential DTIs. It even flagged some promising pairs like D00002 (Nadide) and hsa10935 (Mitochondrial peroxiredoxin3) that could potentially treat neurodegenerative diseases. Now, armed with these discoveries, the researchers set their sights on Parkinson’s and Alzheimer’s disease. EnGDD pointed them towards potential drug targets for each condition – a treasure map waiting to be explored! Although these findings require further validation, the prospects are truly exciting. So grab your pickaxe and delve into the full research article to unravel the secrets of EnGDD!
IntroductionDrug-target interaction prediction is one important step in drug research and development. Experimental methods are time consuming and laborious.MethodsIn this study, we developed a novel DTI prediction method called EnGDD by combining initial feature acquisition, dimensional reduction, and DTI classification based on Gradient boosting neural network, Deep neural network, and Deep Forest.ResultsEnGDD was compared with seven stat-of-the-art DTI prediction methods (BLM-NII, NRLMF, WNNGIP, NEDTP, DTi2Vec, RoFDT, and MolTrans) on the nuclear receptor, GPCR, ion channel, and enzyme datasets under cross validations on drugs, targets, and drug-target pairs, respectively. EnGDD computed the best recall, accuracy, F1-score, AUC, and AUPR under the majority of conditions, demonstrating its powerful DTI identification performance. EnGDD predicted that D00182 and hsa2099, D07871 and hsa1813, DB00599 and hsa2562, D00002 and hsa10935 have a higher interaction probabilities among unknown drug-target pairs and may be potential DTIs on the four datasets, respectively. In particular, D00002 (Nadide) was identified to interact with hsa10935 (Mitochondrial peroxiredoxin3) whose up-regulation might be used to treat neurodegenerative diseases. Finally, EnGDD was used to find possible drug targets for Parkinson’s disease and Alzheimer’s disease after confirming its DTI identification performance. The results show that D01277, D04641, and D08969 may be applied to the treatment of Parkinson’s disease through targeting hsa1813 (dopamine receptor D2) and D02173, D02558, and D03822 may be the clues of treatment for patients with Alzheimer’s disease through targeting hsa5743 (prostaglandinendoperoxide synthase 2). The above prediction results need further biomedical validation.DiscussionWe anticipate that our proposed EnGDD model can help discover potential therapeutic clues for various diseases including neurodegenerative diseases.
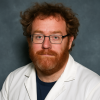
Dr. David Lowemann, M.Sc, Ph.D., is a co-founder of the Institute for the Future of Human Potential, where he leads the charge in pioneering Self-Enhancement Science for the Success of Society. With a keen interest in exploring the untapped potential of the human mind, Dr. Lowemann has dedicated his career to pushing the boundaries of human capabilities and understanding.
Armed with a Master of Science degree and a Ph.D. in his field, Dr. Lowemann has consistently been at the forefront of research and innovation, delving into ways to optimize human performance, cognition, and overall well-being. His work at the Institute revolves around a profound commitment to harnessing cutting-edge science and technology to help individuals lead more fulfilling and intelligent lives.
Dr. Lowemann’s influence extends to the educational platform BetterSmarter.me, where he shares his insights, findings, and personal development strategies with a broader audience. His ongoing mission is shaping the way we perceive and leverage the vast capacities of the human mind, offering invaluable contributions to society’s overall success and collective well-being.