Imagine you’re trying to assemble a complicated puzzle, but you don’t have a picture to guide you. That’s the challenge scientists face when reconstructing the complex neural networks in the brain. However, a recent study has discovered a clever way to overcome this obstacle using a technique called masked autoencoder (MAE) combined with deep learning algorithms. By training an autoencoder to reconstruct neuronal structures from serial scanning electron microscope (SEM) images, researchers were able to uncover the hidden patterns within the brain’s structure. This breakthrough approach has the potential to revolutionize our understanding of the brain’s connectome, which is essential for deciphering how information is processed and transmitted within this intricate organ. If you’re curious to learn more about this exciting research and its implications for neuroscience, dive into the full article!
IntroductionThe exorbitant cost of accurately annotating the large-scale serial scanning electron microscope (SEM) images as the ground truth for training has always been a great challenge for brain map reconstruction by deep learning methods in neural connectome studies. The representation ability of the model is strongly correlated with the number of such high-quality labels. Recently, the masked autoencoder (MAE) has been shown to effectively pre-train Vision Transformers (ViT) to improve their representational capabilities.MethodsIn this paper, we investigated a self-pre-training paradigm for serial SEM images with MAE to implement downstream segmentation tasks. We randomly masked voxels in three-dimensional brain image patches and trained an autoencoder to reconstruct the neuronal structures.Results and discussionWe tested different pre-training and fine-tuning configurations on three different serial SEM datasets of mouse brains, including two public ones, SNEMI3D and MitoEM-R, and one acquired in our lab. A series of masking ratios were examined and the optimal ratio for pre-training efficiency was spotted for 3D segmentation. The MAE pre-training strategy significantly outperformed the supervised learning from scratch. Our work shows that the general framework of can be a unified approach for effective learning of the representation of heterogeneous neural structural features in serial SEM images to greatly facilitate brain connectome reconstruction.
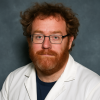
Dr. David Lowemann, M.Sc, Ph.D., is a co-founder of the Institute for the Future of Human Potential, where he leads the charge in pioneering Self-Enhancement Science for the Success of Society. With a keen interest in exploring the untapped potential of the human mind, Dr. Lowemann has dedicated his career to pushing the boundaries of human capabilities and understanding.
Armed with a Master of Science degree and a Ph.D. in his field, Dr. Lowemann has consistently been at the forefront of research and innovation, delving into ways to optimize human performance, cognition, and overall well-being. His work at the Institute revolves around a profound commitment to harnessing cutting-edge science and technology to help individuals lead more fulfilling and intelligent lives.
Dr. Lowemann’s influence extends to the educational platform BetterSmarter.me, where he shares his insights, findings, and personal development strategies with a broader audience. His ongoing mission is shaping the way we perceive and leverage the vast capacities of the human mind, offering invaluable contributions to society’s overall success and collective well-being.