Imagine you’re trying to take a picture of a moving object. The object is constantly changing, so you want your camera to capture the details without getting blurry. Well, it turns out that our brains face a similar challenge when processing dynamic visual stimuli. To optimize efficiency, neural representations try to minimize redundant information, but this can make them vulnerable to random noise. One way to deal with this is by smoothing neural responses, which helps protect against both noise and temporal delays. A recent study explored whether this smoothness can maintain robust neural representations in hierarchical brain structures. Using simulations, researchers demonstrated that a hierarchical neural network trained with smooth coding could produce similar responses for moving and static visual stimuli. This suggests that smooth coding preserves the structure of visual environments in hierarchical brain networks. These findings emphasize the importance of finding a balance between efficiency and robustness in neural coding for processing dynamic visual stimuli in the brain. To dive into the details, check out the full article!
IntroductionEfficient coding that minimizes informational redundancy of neural representations is a widely accepted neural coding principle. Despite the benefit, maximizing efficiency in neural coding can make neural representation vulnerable to random noise. One way to achieve robustness against random noise is smoothening neural responses. However, it is not clear whether the smoothness of neural responses can hold robust neural representations when dynamic stimuli are processed through a hierarchical brain structure, in which not only random noise but also systematic error due to temporal lag can be induced.MethodsIn the present study, we showed that smoothness via spatio-temporally efficient coding can achieve both efficiency and robustness by effectively dealing with noise and neural delay in the visual hierarchy when processing dynamic visual stimuli.ResultsThe simulation results demonstrated that a hierarchical neural network whose bidirectional synaptic connections were learned through spatio-temporally efficient coding with natural scenes could elicit neural responses to visual moving bars similar to those to static bars with the identical position and orientation, indicating robust neural responses against erroneous neural information. It implies that spatio-temporally efficient coding preserves the structure of visual environments locally in the neural responses of hierarchical structures.DiscussionThe present results suggest the importance of a balance between efficiency and robustness in neural coding for visual processing of dynamic stimuli across hierarchical brain structures.
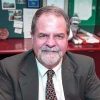
Dr. David Lowemann, M.Sc, Ph.D., is a co-founder of the Institute for the Future of Human Potential, where he leads the charge in pioneering Self-Enhancement Science for the Success of Society. With a keen interest in exploring the untapped potential of the human mind, Dr. Lowemann has dedicated his career to pushing the boundaries of human capabilities and understanding.
Armed with a Master of Science degree and a Ph.D. in his field, Dr. Lowemann has consistently been at the forefront of research and innovation, delving into ways to optimize human performance, cognition, and overall well-being. His work at the Institute revolves around a profound commitment to harnessing cutting-edge science and technology to help individuals lead more fulfilling and intelligent lives.
Dr. Lowemann’s influence extends to the educational platform BetterSmarter.me, where he shares his insights, findings, and personal development strategies with a broader audience. His ongoing mission is shaping the way we perceive and leverage the vast capacities of the human mind, offering invaluable contributions to society’s overall success and collective well-being.