In the quest to understand Alzheimer’s disease (AD), scientists have turned their attention to a common RNA chemical modification called m7G. This modification plays a role in the development of many diseases, including AD. By analyzing gene expression data and immune signatures, researchers identified m7G-related AD subtypes and developed a machine learning model to predict these subtypes. The study found that patients with AD had dysregulated m7G genes compared to non-AD patients, indicating immune differences between the two groups. Using the differentially expressed m7G regulators, AD patients were classified into two clusters, with Cluster 2 showing higher immune infiltration. The best-performing predictive model was the Random Forest model, which achieved an impressive AUC value of 1.000. The model’s accuracy was further validated using an external dataset, obtaining an AUC value of 0.968. This research sheds light on the biological significance of m7G methylation in AD and highlights its association with immune characteristics. It also presents a potential tool for risk assessment and management of AD patients. To dive deeper into this groundbreaking study, check out the full article!
IntroductionAlzheimer’s disease (AD) is a complex and progressive neurodegenerative disorder that primarily affects older individuals. N7-methylguanosine (m7G) is a common RNA chemical modification that impacts the development of numerous diseases. Thus, our work investigated m7G-related AD subtypes and established a predictive model.MethodsThe datasets for AD patients, including GSE33000 and GSE44770, were obtained from the Gene Expression Omnibus (GEO) database, which were derived from the prefrontal cortex of the brain. We performed differential analysis of m7G regulators and examined the immune signatures differences between AD and matched-normal samples. Consensus clustering was employed to identify AD subtypes based on m7G-related differentially expressed genes (DEGs), and immune signatures were explored among different clusters. Furthermore, we developed four machine learning models based on the expression profiles of m7G-related DEGs and identified five important genes from the optimal model. We evaluated the predictive power of the 5-gene-based model using an external AD dataset (GSE44770).ResultsA total of 15 genes related to m7G were found to be dysregulated in patients with AD compared to non-AD patients. This finding suggests that there are differences in immune characteristics between these two groups. Based on the differentially expressed m7G regulators, we categorized AD patients into two clusters and calculated the ESTIMATE score for each cluster. Cluster 2 exhibited a higher ImmuneScore than Cluster 1. We performed the receiver operating characteristic (ROC) analysis to compare the performance of four models, and we found that the Random Forest (RF) model had the highest AUC value of 1.000. Furthermore, we tested the predictive efficacy of a 5-gene-based RF model on an external AD dataset and obtained an AUC value of 0.968. The nomogram, calibration curve, and decision curve analysis (DCA) confirmed the accuracy of our model in predicting AD subtypes.ConclusionThe present study systematically examines the biological significance of m7G methylation modification in AD and investigates its association with immune infiltration characteristics. Furthermore, the study develops potential predictive models to assess the risk of m7G subtypes and the pathological outcomes of patients with AD, which can facilitate risk classification and clinical management of AD patients.
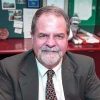
Dr. David Lowemann, M.Sc, Ph.D., is a co-founder of the Institute for the Future of Human Potential, where he leads the charge in pioneering Self-Enhancement Science for the Success of Society. With a keen interest in exploring the untapped potential of the human mind, Dr. Lowemann has dedicated his career to pushing the boundaries of human capabilities and understanding.
Armed with a Master of Science degree and a Ph.D. in his field, Dr. Lowemann has consistently been at the forefront of research and innovation, delving into ways to optimize human performance, cognition, and overall well-being. His work at the Institute revolves around a profound commitment to harnessing cutting-edge science and technology to help individuals lead more fulfilling and intelligent lives.
Dr. Lowemann’s influence extends to the educational platform BetterSmarter.me, where he shares his insights, findings, and personal development strategies with a broader audience. His ongoing mission is shaping the way we perceive and leverage the vast capacities of the human mind, offering invaluable contributions to society’s overall success and collective well-being.