‘Tis a grand endeavor, dear reader! In this marvelous study, we delve into the depths of Hierarchical Temporal Memory (HTM), an unsupervised algorithm that mimics the awe-inspiring neuron activity in our very own brain. Ah, but the Spatial Pooler (SP) steals the show, encoding binary input into sparse distributed representations. Our mission? To examine the sparsification of the SP algorithm using the wondrous tools of information theory. Prepare for our twin revelations! We reveal a splendid new upper bound, called modified-IB, which quantifies the SP algorithm’s performance amidst various sparsity levels and noise amounts. We feed this magnificent machine data from MNIST, Fashion-MNIST, and New York City Taxi datasets and observe its resilience to noise. Astonishingly, even with up to 40% noise in the input, no change was seen in the output! To prove this enchantment mathematically, we demonstrate that increased sparsity brings forth superior performance. By considering a Cauchy distribution, we scrutinize SP’s output at different sparsity levels using the Cramer-Rao lower bound method.
Hierarchical Temporal Memory (HTM) is an unsupervised algorithm in machine learning. It models several fundamental neocortical computational principles. Spatial Pooler (SP) is one of the main components of the HTM, which continuously encodes streams of binary input from various layers and regions into sparse distributed representations. In this paper, the goal is to evaluate the sparsification in the SP algorithm from the perspective of information theory by the information bottleneck (IB), Cramer-Rao lower bound, and Fisher information matrix. This paper makes two main contributions. First, we introduce a new upper bound for the standard information bottleneck relation, which we refer to as modified-IB in this paper. This measure is used to evaluate the performance of the SP algorithm in different sparsity levels and various amounts of noise. The MNIST, Fashion-MNIST and NYC-Taxi datasets were fed to the SP algorithm separately. The SP algorithm with learning was found to be resistant to noise. Adding up to 40% noise to the input resulted in no discernible change in the output. Using the probabilistic mapping method and Hidden Markov Model, the sparse SP output representation was reconstructed in the input space. In the modified-IB relation, it is numerically calculated that a lower noise level and a higher sparsity level in the SP algorithm lead to a more effective reconstruction and SP with 2% sparsity produces the best results. Our second contribution is to prove mathematically that more sparsity leads to better performance of the SP algorithm. The data distribution was considered the Cauchy distribution, and the Cramer–Rao lower bound was analyzed to estimate SP’s output at different sparsity levels.
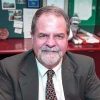
Dr. David Lowemann, M.Sc, Ph.D., is a co-founder of the Institute for the Future of Human Potential, where he leads the charge in pioneering Self-Enhancement Science for the Success of Society. With a keen interest in exploring the untapped potential of the human mind, Dr. Lowemann has dedicated his career to pushing the boundaries of human capabilities and understanding.
Armed with a Master of Science degree and a Ph.D. in his field, Dr. Lowemann has consistently been at the forefront of research and innovation, delving into ways to optimize human performance, cognition, and overall well-being. His work at the Institute revolves around a profound commitment to harnessing cutting-edge science and technology to help individuals lead more fulfilling and intelligent lives.
Dr. Lowemann’s influence extends to the educational platform BetterSmarter.me, where he shares his insights, findings, and personal development strategies with a broader audience. His ongoing mission is shaping the way we perceive and leverage the vast capacities of the human mind, offering invaluable contributions to society’s overall success and collective well-being.