Just like a two-part dance routine, this study introduces a neural network model that classifies the initial stages of Parkinson’s disease. The first stage uses semantic segmentation to analyze the sensor signals and gather valuable biomechanical variables. In the second stage, a multidimensional neural network takes input from the biomechanical variables, spectrogram image, and raw sensor signals. With impressive accuracy rates of 99.64% during training/validation and a perfect success rate in the test phase, this model shows great promise for identifying Parkinson’s disease stages. By using a simple 2-minute functional test that requires minimal instrumentation and is easy to administer, healthcare professionals can swiftly intervene and improve patient outcomes. Now it’s time to dive deeper into this groundbreaking research!
IntroductionParkinson’s disease is one of the most prevalent neurodegenerative diseases. In the most advanced stages, PD produces motor dysfunction that impairs basic activities of daily living such as balance, gait, sitting, or standing. Early identification allows healthcare personnel to intervene more effectively in rehabilitation. Understanding the altered aspects and impact on the progression of the disease is important for improving the quality of life. This study proposes a two-stage neural network model for the classifying the initial stages of PD using data recorded with smartphone sensors during a modified Timed Up & Go test.MethodsThe proposed model consists on two stages: in the first stage, a semantic segmentation of the raw sensor signals classifies the activities included in the test and obtains biomechanical variables that are considered clinically relevant parameters for functional assessment. The second stage is a neural network with three input branches: one with the biomechanical variables, one with the spectrogram image of the sensor signals, and the third with the raw sensor signals.ResultsThis stage employs convolutional layers and long short-term memory. The results show a mean accuracy of 99.64% for the stratified k-fold training/validation process and 100% success rate of participants in the test phase.DiscussionThe proposed model is capable of identifying the three initial stages of Parkinson’s disease using a 2-min functional test. The test easy instrumentation requirements and short duration make it feasible for use feasible in the clinical context.
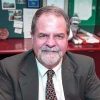
Dr. David Lowemann, M.Sc, Ph.D., is a co-founder of the Institute for the Future of Human Potential, where he leads the charge in pioneering Self-Enhancement Science for the Success of Society. With a keen interest in exploring the untapped potential of the human mind, Dr. Lowemann has dedicated his career to pushing the boundaries of human capabilities and understanding.
Armed with a Master of Science degree and a Ph.D. in his field, Dr. Lowemann has consistently been at the forefront of research and innovation, delving into ways to optimize human performance, cognition, and overall well-being. His work at the Institute revolves around a profound commitment to harnessing cutting-edge science and technology to help individuals lead more fulfilling and intelligent lives.
Dr. Lowemann’s influence extends to the educational platform BetterSmarter.me, where he shares his insights, findings, and personal development strategies with a broader audience. His ongoing mission is shaping the way we perceive and leverage the vast capacities of the human mind, offering invaluable contributions to society’s overall success and collective well-being.