Imagine trying to navigate a complex and noisy video game without any prior knowledge or guidance. You rely on your intuition and experiences to make sense of patterns, formulate predictions, and build a mental framework. In a similar way, humans learn the regularities of their world through unsupervised interactions with the environment. But what fuels the acquisition of hierarchical spatiotemporal concepts? Researchers propose that the desire to improve predictions may play a significant role in this process. To explore this hypothesis, they introduce an information-theoretic score called CORE. This score evaluates how well concepts fit their context and align with reality. By driving learners to construct larger and more accurate concepts, CORE guides the development of hierarchical representations and paves the way for efficient learning and organization of knowledge.
The researchers implement this approach in a prediction game framework, starting from basic concepts like individual characters and gradually building a networked hierarchy of concepts over time. They demonstrate that CORE is scalable and open-ended, enabling the learning of thousands of concepts across hundreds of thousands of episodes. The system’s performance is compared with transformer neural networks and n-gram language models, providing insights into its strengths and differences compared to existing techniques.
This study sheds light on the cognitive processes behind hierarchical concept acquisition and offers valuable implications for fields like artificial intelligence and machine learning. To dive deeper into the research and explore potential future directions, check out the full article!
How do humans learn the regularities of their complex noisy world in a robust manner? There is ample evidence that much of this learning and development occurs in an unsupervised fashion via interactions with the environment. Both the structure of the world as well as the brain appear hierarchical in a number of ways, and structured hierarchical representations offer potential benefits for efficient learning and organization of knowledge, such as concepts (patterns) sharing parts (subpatterns), and for providing a foundation for symbolic computation and language. A major question arises: what drives the processes behind acquiring such hierarchical spatiotemporal concepts? We posit that the goal of advancing one’s predictions is a major driver for learning such hierarchies and introduce an information-theoretic score that shows promise in guiding the processes, and, in particular, motivating the learner to build larger concepts. We have been exploring the challenges of building an integrated learning and developing system within the framework of prediction games, wherein concepts serve as (1) predictors, (2) targets of prediction, and (3) building blocks for future higher-level concepts. Our current implementation works on raw text: it begins at a low level, such as characters, which are the hardwired or primitive concepts, and grows its vocabulary of networked hierarchical concepts over time. Concepts are strings or n-grams in our current realization, but we hope to relax this limitation, e.g., to a larger subclass of finite automata. After an overview of the current system, we focus on the score, named CORE. CORE is based on comparing the prediction performance of the system with a simple baseline system that is limited to predicting with the primitives. CORE incorporates a tradeoff between how strongly a concept is predicted (or how well it fits its context, i.e., nearby predicted concepts) vs. how well it matches the (ground) “reality,” i.e., the lowest level observations (the characters in the input episode). CORE is applicable to generative models such as probabilistic finite state machines (beyond strings). We highlight a few properties of CORE with examples. The learning is scalable and open-ended. For instance, thousands of concepts are learned after hundreds of thousands of episodes. We give examples of what is learned, and we also empirically compare with transformer neural networks and n-gram language models to situate the current implementation with respect to state-of-the-art and to further illustrate the similarities and differences with existing techniques. We touch on a variety of challenges and promising future directions in advancing the approach, in particular, the challenge of learning concepts with a more sophisticated structure.
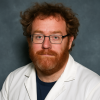
Dr. David Lowemann, M.Sc, Ph.D., is a co-founder of the Institute for the Future of Human Potential, where he leads the charge in pioneering Self-Enhancement Science for the Success of Society. With a keen interest in exploring the untapped potential of the human mind, Dr. Lowemann has dedicated his career to pushing the boundaries of human capabilities and understanding.
Armed with a Master of Science degree and a Ph.D. in his field, Dr. Lowemann has consistently been at the forefront of research and innovation, delving into ways to optimize human performance, cognition, and overall well-being. His work at the Institute revolves around a profound commitment to harnessing cutting-edge science and technology to help individuals lead more fulfilling and intelligent lives.
Dr. Lowemann’s influence extends to the educational platform BetterSmarter.me, where he shares his insights, findings, and personal development strategies with a broader audience. His ongoing mission is shaping the way we perceive and leverage the vast capacities of the human mind, offering invaluable contributions to society’s overall success and collective well-being.