Imagine you have a bunch of words and you want to understand how they relate to each other. Well, that’s exactly what scientists do with distributional semantic models (DSMs)! These models use fancy math to analyze big collections of text and figure out the hidden meanings behind words. But there’s still something scientists want to know: What kinds of relationships can DSMs detect? To find out, researchers tested eight popular DSMs and discovered some interesting results. It turns out that DSMs are really good at capturing semantic similarities between words. They’re also pretty adept at picking up on connections between verbs and nouns, as well as between different types of events. But each DSM has its own strengths, with Skip-gram and CBOW leading the way in similarity detection, while GloVe shines when it comes to thematic role relations and event-based relations. These findings have big implications for how we use these models, and they show us that not all DSMs are created equal! If you’re interested in unlocking the power of distributional semantic models, check out the full article.
Abstract
Distributional semantic models (DSMs) are a primary method for distilling semantic information from corpora. However, a key question remains: What types of semantic relations among words do DSMs detect? Prior work typically has addressed this question using limited human data that are restricted to semantic similarity and/or general semantic relatedness. We tested eight DSMs that are popular in current cognitive and psycholinguistic research (positive pointwise mutual information; global vectors; and three variations each of Skip-gram and continuous bag of words (CBOW) using word, context, and mean embeddings) on a theoretically motivated, rich set of semantic relations involving words from multiple syntactic classes and spanning the abstract–concrete continuum (19 sets of ratings). We found that, overall, the DSMs are best at capturing overall semantic similarity and also can capture verb–noun thematic role relations and noun–noun event-based relations that play important roles in sentence comprehension. Interestingly, Skip-gram and CBOW performed the best in terms of capturing similarity, whereas GloVe dominated the thematic role and event-based relations. We discuss the theoretical and practical implications of our results, make recommendations for users of these models, and demonstrate significant differences in model performance on event-based relations.
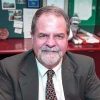
Dr. David Lowemann, M.Sc, Ph.D., is a co-founder of the Institute for the Future of Human Potential, where he leads the charge in pioneering Self-Enhancement Science for the Success of Society. With a keen interest in exploring the untapped potential of the human mind, Dr. Lowemann has dedicated his career to pushing the boundaries of human capabilities and understanding.
Armed with a Master of Science degree and a Ph.D. in his field, Dr. Lowemann has consistently been at the forefront of research and innovation, delving into ways to optimize human performance, cognition, and overall well-being. His work at the Institute revolves around a profound commitment to harnessing cutting-edge science and technology to help individuals lead more fulfilling and intelligent lives.
Dr. Lowemann’s influence extends to the educational platform BetterSmarter.me, where he shares his insights, findings, and personal development strategies with a broader audience. His ongoing mission is shaping the way we perceive and leverage the vast capacities of the human mind, offering invaluable contributions to society’s overall success and collective well-being.