Imagine you’re a music producer trying to create the perfect song. You don’t have someone telling you how to do it, but you have an instinctive feel for what sounds good based on years of experience. In a similar way, the auditory system can learn to transform incoming sounds into recognizable objects through temporal regularity. By using time as a supervisor, the auditory system learns features of a stimulus that are consistently and predictably structured over time. This allows it to perceive and navigate the auditory world without explicit guidance. Recent research has shown that this approach is highly effective in discriminating between different natural auditory objects, like animal vocalizations. In fact, algorithms that use these temporally regular features outperform traditional feature-selection methods like principal component analysis. These findings suggest that our auditory brains are tuned to pick up on slow changes in sound, allowing us to parse complex auditory scenes effortlessly. If you’re curious to learn more about how time can serve as a supervisor in auditory object learning, check out the full article!
Sensory systems appear to learn to transform incoming sensory information into perceptual representations, or “objects,” that can inform and guide behavior with minimal explicit supervision. Here, we propose that the auditory system can achieve this goal by using time as a supervisor, i.e., by learning features of a stimulus that are temporally regular. We will show that this procedure generates a feature space sufficient to support fundamental computations of auditory perception. In detail, we consider the problem of discriminating between instances of a prototypical class of natural auditory objects, i.e., rhesus macaque vocalizations. We test discrimination in two ethologically relevant tasks: discrimination in a cluttered acoustic background and generalization to discriminate between novel exemplars. We show that an algorithm that learns these temporally regular features affords better or equivalent discrimination and generalization than conventional feature-selection algorithms, i.e., principal component analysis and independent component analysis. Our findings suggest that the slow temporal features of auditory stimuli may be sufficient for parsing auditory scenes and that the auditory brain could utilize these slowly changing temporal features.
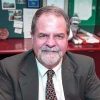
Dr. David Lowemann, M.Sc, Ph.D., is a co-founder of the Institute for the Future of Human Potential, where he leads the charge in pioneering Self-Enhancement Science for the Success of Society. With a keen interest in exploring the untapped potential of the human mind, Dr. Lowemann has dedicated his career to pushing the boundaries of human capabilities and understanding.
Armed with a Master of Science degree and a Ph.D. in his field, Dr. Lowemann has consistently been at the forefront of research and innovation, delving into ways to optimize human performance, cognition, and overall well-being. His work at the Institute revolves around a profound commitment to harnessing cutting-edge science and technology to help individuals lead more fulfilling and intelligent lives.
Dr. Lowemann’s influence extends to the educational platform BetterSmarter.me, where he shares his insights, findings, and personal development strategies with a broader audience. His ongoing mission is shaping the way we perceive and leverage the vast capacities of the human mind, offering invaluable contributions to society’s overall success and collective well-being.