Imagine you’re trying to decipher a message written in code, and the code keeps changing. This is similar to the challenge faced by researchers developing brain-computer interfaces (BCIs) that allow paralyzed individuals to control devices with their thoughts. In a simulation study, scientists explored how the non-stationarity of spike signals, caused by recording degradation and variations in neuronal properties, affects the performance of BCIs. They used various metrics, such as mean firing rate and neural preferred directions, to simulate these signal changes. Three different decoders were tested, and two training schemes were implemented. The results showed that a specific decoder, called Recurrent Neural Network (RNN), performed consistently better when the recording degradation was minimal. However, severe signal degradation led to a decline in performance for all decoders. Interestingly, the RNN decoder outperformed the other two decoders when it came to decoding simulated non-stationary spike signals. Moreover, using a retrained training scheme helped maintain high performance when only changes in neuronal properties were limited. This study provides valuable insights into selecting appropriate decoders and training schemes for BCIs in real-world scenarios where signal non-stationarities are present. To delve deeper into this topic, check out the underlying research!
IntroductionIntracortical Brain-Computer Interfaces (iBCI) establish a new pathway to restore motor functions in individuals with paralysis by interfacing directly with the brain to translate movement intention into action. However, the development of iBCI applications is hindered by the non-stationarity of neural signals induced by the recording degradation and neuronal property variance. Many iBCI decoders were developed to overcome this non-stationarity, but its effect on decoding performance remains largely unknown, posing a critical challenge for the practical application of iBCI.MethodsTo improve our understanding on the effect of non-stationarity, we conducted a 2D-cursor simulation study to examine the influence of various types of non-stationarities. Concentrating on spike signal changes in chronic intracortical recording, we used the following three metrics to simulate the non-stationarity: mean firing rate (MFR), number of isolated units (NIU), and neural preferred directions (PDs). MFR and NIU were decreased to simulate the recording degradation while PDs were changed to simulate the neuronal property variance. Performance evaluation based on simulation data was then conducted on three decoders and two different training schemes. Optimal Linear Estimation (OLE), Kalman Filter (KF), and Recurrent Neural Network (RNN) were implemented as decoders and trained using static and retrained schemes.ResultsIn our evaluation, RNN decoder and retrained scheme showed consistent better performance under small recording degradation. However, the serious signal degradation would cause significant performance to drop eventually. On the other hand, RNN performs significantly better than the other two decoders in decoding simulated non-stationary spike signals, and the retrained scheme maintains the decoders’ high performance when changes are limited to PDs.DiscussionOur simulation work demonstrates the effects of neural signal non-stationarity on decoding performance and serves as a reference for selecting decoders and training schemes in chronic iBCI. Our result suggests that comparing to KF and OLE, RNN has better or equivalent performance using both training schemes. Performance of decoders under static scheme is influenced by recording degradation and neuronal property variation while decoders under retrained scheme are only influenced by the former one.
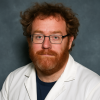
Dr. David Lowemann, M.Sc, Ph.D., is a co-founder of the Institute for the Future of Human Potential, where he leads the charge in pioneering Self-Enhancement Science for the Success of Society. With a keen interest in exploring the untapped potential of the human mind, Dr. Lowemann has dedicated his career to pushing the boundaries of human capabilities and understanding.
Armed with a Master of Science degree and a Ph.D. in his field, Dr. Lowemann has consistently been at the forefront of research and innovation, delving into ways to optimize human performance, cognition, and overall well-being. His work at the Institute revolves around a profound commitment to harnessing cutting-edge science and technology to help individuals lead more fulfilling and intelligent lives.
Dr. Lowemann’s influence extends to the educational platform BetterSmarter.me, where he shares his insights, findings, and personal development strategies with a broader audience. His ongoing mission is shaping the way we perceive and leverage the vast capacities of the human mind, offering invaluable contributions to society’s overall success and collective well-being.