Imagine you’re at a cooking competition, where the chefs have limited resources and are trying to process a huge pile of ingredients. In this scenario, they could either drag all the ingredients back to their kitchen for processing or find a way to process them right on the spot. Similarly, edge devices equipped with computer vision face the challenge of dealing with vast amounts of sensory data using their limited computing resources. To tackle this issue, scientists have been exploring energy-efficient solutions that bring the computation closer to the sensor. One such solution is in-pixel processing, which embeds the computation capabilities inside the pixel array of CMOS image sensors. However, until now, the processing-in-pixel approach for neuromorphic vision sensors remained unexplored.
Now, for the first time ever, researchers have proposed an innovative technique called Neuromorphic-P2M (Processing-in-Pixel-in-Memory) paradigm. This asynchronous non-von-Neumann analog processing-in-pixel paradigm enables convolution operations inside the pixel array, resulting in high energy efficiency with significantly lower energy consumption compared to traditional digital methods. The researchers developed a hardware-algorithm co-design framework that combines circuit designs and algorithms to account for imperfections, leakage, and variations in the circuit’s behavior. They used extensive simulations with GF22nm FD-SOI technology and verified their approach using state-of-the-art neuromorphic vision sensor datasets.
Notably, their solution achieved approximately 2 times lower backend-processor energy consumption while maintaining similar front-end (sensor) energy compared to existing techniques on the IBM DVS128-Gesture dataset. It also demonstrated a high test accuracy of 88.36%. This groundbreaking research opens up exciting possibilities for revolutionizing image sensor processing and enhancing energy efficiency in various applications such as computer vision and edge computing. To learn more about the details and implications of this research, check out the full article!
Edge devices equipped with computer vision must deal with vast amounts of sensory data with limited computing resources. Hence, researchers have been exploring different energy-efficient solutions such as near-sensor, in-sensor, and in-pixel processing, bringing the computation closer to the sensor. In particular, in-pixel processing embeds the computation capabilities inside the pixel array and achieves high energy efficiency by generating low-level features instead of the raw data stream from CMOS image sensors. Many different in-pixel processing techniques and approaches have been demonstrated on conventional frame-based CMOS imagers; however, the processing-in-pixel approach for neuromorphic vision sensors has not been explored so far. In this work, for the first time, we propose an asynchronous non-von-Neumann analog processing-in-pixel paradigm to perform convolution operations by integrating in-situ multi-bit multi-channel convolution inside the pixel array performing analog multiply and accumulate (MAC) operations that consume significantly less energy than their digital MAC alternative. To make this approach viable, we incorporate the circuit’s non-ideality, leakage, and process variations into a novel hardware-algorithm co-design framework that leverages extensive HSpice simulations of our proposed circuit using the GF22nm FD-SOI technology node. We verified our framework on state-of-the-art neuromorphic vision sensor datasets and show that our solution consumes ~2× lower backend-processor energy while maintaining almost similar front-end (sensor) energy on the IBM DVS128-Gesture dataset than the state-of-the-art while maintaining a high test accuracy of 88.36%.
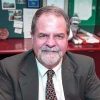
Dr. David Lowemann, M.Sc, Ph.D., is a co-founder of the Institute for the Future of Human Potential, where he leads the charge in pioneering Self-Enhancement Science for the Success of Society. With a keen interest in exploring the untapped potential of the human mind, Dr. Lowemann has dedicated his career to pushing the boundaries of human capabilities and understanding.
Armed with a Master of Science degree and a Ph.D. in his field, Dr. Lowemann has consistently been at the forefront of research and innovation, delving into ways to optimize human performance, cognition, and overall well-being. His work at the Institute revolves around a profound commitment to harnessing cutting-edge science and technology to help individuals lead more fulfilling and intelligent lives.
Dr. Lowemann’s influence extends to the educational platform BetterSmarter.me, where he shares his insights, findings, and personal development strategies with a broader audience. His ongoing mission is shaping the way we perceive and leverage the vast capacities of the human mind, offering invaluable contributions to society’s overall success and collective well-being.