Imagine you’re building a puzzle. One approach is to focus on individual puzzle pieces and their relationships, while another approach is to identify larger, cohesive sections. These two approaches also exist in the field of statistical learning, which explores how we extract patterns from sequences. The transitional probability approach emphasizes the computation of probabilities between items in a sequence, similar to focusing on individual puzzle pieces. On the other hand, the chunking approach suggests that we extract full units, like identifying larger puzzle sections. In a fascinating study, researchers compared these two approaches using visual stimuli and an online self-paced task. They discovered that when participants were presented with altered triplets, which disrupted the full unit while preserving a subunit, processing for subunits was impeded. This finding supports the chunking approach to statistical learning and provides insights into how we perceive and process information. To delve deeper into the research and uncover the intricacies of statistical learning theories, check out the full article!
Abstract
There are two main approaches to how statistical patterns are extracted from sequences: The transitional probability approach proposes that statistical learning occurs through the computation of probabilities between items in a sequence. The chunking approach, including models such as PARSER and TRACX, proposes that units are extracted as chunks. Importantly, the chunking approach suggests that the extraction of full units weakens the processing of subunits while the transitional probability approach suggests that both units and subunits should strengthen. Previous findings using sequentially organized, auditory stimuli or spatially organized, visual stimuli support the chunking approach. However, one limitation of prior studies is that most assessed learning with the two-alternative forced-choice task. In contrast, this pre-registered experiment examined the two theoretical approaches in sequentially organized, visual stimuli using an online self-paced task—arguably providing a more sensitive index of learning as it occurs—and a secondary offline familiarity judgment task. During the self-paced task, abstract shapes were covertly organized into eight triplets (ABC) where one in every eight was altered (BCA) from the canonical structure in a way that disrupted the full unit while preserving a subunit (BC). Results from the offline familiarity judgment task revealed that the altered triplets were perceived as highly familiar, suggesting the learned representations were relatively flexible. More importantly, results from the online self-paced task demonstrated that processing for subunits, but not unit-initial stimuli, was impeded in the altered triplet. The pattern of results is in line with the chunking approach to statistical learning and, more specifically, the TRACX model.
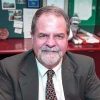
Dr. David Lowemann, M.Sc, Ph.D., is a co-founder of the Institute for the Future of Human Potential, where he leads the charge in pioneering Self-Enhancement Science for the Success of Society. With a keen interest in exploring the untapped potential of the human mind, Dr. Lowemann has dedicated his career to pushing the boundaries of human capabilities and understanding.
Armed with a Master of Science degree and a Ph.D. in his field, Dr. Lowemann has consistently been at the forefront of research and innovation, delving into ways to optimize human performance, cognition, and overall well-being. His work at the Institute revolves around a profound commitment to harnessing cutting-edge science and technology to help individuals lead more fulfilling and intelligent lives.
Dr. Lowemann’s influence extends to the educational platform BetterSmarter.me, where he shares his insights, findings, and personal development strategies with a broader audience. His ongoing mission is shaping the way we perceive and leverage the vast capacities of the human mind, offering invaluable contributions to society’s overall success and collective well-being.