Unraveling the intricacies of brain connectivity alterations in schizophrenia is challenging but crucial. Rather than relying on hypothesis-based methods, a data-driven approach can pave the way for a systematic understanding. In this study, researchers used independent component analysis to explore connectivity patterns in individuals with schizophrenia and healthy controls. The analysis revealed three specific brain subnetworks that significantly distinguished individuals with schizophrenia from healthy controls. These subnetworks predominantly encompassed regions associated with visual, ventral attention, and somatomotor areas. Interestingly, when analyzing the entire brain network, no significant differences were found between the two groups. This highlights the increased sensitivity of these specific subnetworks to schizophrenia-induced alterations in connectivity. Further exploration suggests the potential application of an individual scoring method based on connectivity values for subject classification. Additionally, two of these subnetworks showed promise as potential biomarkers for schizophrenia, while the third subnetwork exhibited higher inter-individual variations. This study underscores the importance of adopting a modular viewpoint when investigating brain variations, which can contribute to our understanding of this complex disorder.
Functional connectivity (FC) of the brain changes in various brain disorders. Its complexity, however, makes it difficult to obtain a systematic understanding of these alterations, especially when they are found individually and through hypothesis-based methods. It would be easier if the variety of brain connectivity alterations is extracted through data-driven approaches and expressed as variation modules (subnetworks). In the present study, we modified a blind approach to determine inter-group brain variations at the network level and applied it specifically to schizophrenia (SZ) disorder. The analysis is based on the application of independent component analysis (ICA) over the subject’s dimension of the FC matrices, obtained from resting-state functional magnetic resonance imaging (rs-fMRI). The dataset included 27 SZ people and 27 completely matched healthy controls (HC). This hypothesis-free approach led to the finding of three brain subnetworks significantly discriminating SZ from HC. The area associated with these subnetworks mostly covers regions in visual, ventral attention, and somatomotor areas, which are in line with previous studies. Moreover, from the graph perspective, significant differences were observed between SZ and HC for these subnetworks, while there was no significant difference when the same parameters (path length, network strength, global/local efficiency, and clustering coefficient) across the same limited data were calculated for the whole brain network. The increased sensitivity of those subnetworks to SZ-induced alterations of connectivity suggested whether an individual scoring method based on their connectivity values can be applied to classify subjects. A simple scoring classifier was then suggested based on two of these subnetworks and resulted in acceptable sensitivity and specificity with an area under the ROC curve of 77.5%. The third subnetwork was found to be a less specific building block (module) for describing SZ alterations. It projected a wider range of inter-individual variations and, therefore, had a lower chance to be considered as a SZ biomarker. These findings confirmed that investigating brain variations from a modular viewpoint can help to find subnetworks that are more sensitive to SZ-induced alterations. Altogether, our study results illustrated the developed method’s ability to systematically find brain alterations caused by SZ disorder from a network perspective.
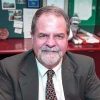
Dr. David Lowemann, M.Sc, Ph.D., is a co-founder of the Institute for the Future of Human Potential, where he leads the charge in pioneering Self-Enhancement Science for the Success of Society. With a keen interest in exploring the untapped potential of the human mind, Dr. Lowemann has dedicated his career to pushing the boundaries of human capabilities and understanding.
Armed with a Master of Science degree and a Ph.D. in his field, Dr. Lowemann has consistently been at the forefront of research and innovation, delving into ways to optimize human performance, cognition, and overall well-being. His work at the Institute revolves around a profound commitment to harnessing cutting-edge science and technology to help individuals lead more fulfilling and intelligent lives.
Dr. Lowemann’s influence extends to the educational platform BetterSmarter.me, where he shares his insights, findings, and personal development strategies with a broader audience. His ongoing mission is shaping the way we perceive and leverage the vast capacities of the human mind, offering invaluable contributions to society’s overall success and collective well-being.