Just like a detective deciphering clues, information theory allows us to unravel the mysteries of the brain’s information processing. It’s like having a versatile tool that can make sense of complex brain data, regardless of its structure. By using information-theoretical metrics, such as Entropy and Mutual Information, we can gain valuable insights into neurophysiological recordings. But how do these metrics stack up against the trusty t-test? In this study, researchers take on the challenge of comparing the performance of different methods, including Encoded Information with Mutual Information, Gaussian Copula Mutual Information, Neural Frequency Tagging, and the t-test. They apply these methods to data collected from human and marmoset monkey electroencephalography (EEG) recordings, specifically event-related potentials and activity in different frequency bands. Encoded Information is a particularly fascinating new approach that examines the similarity of brain responses across conditions by compressing the signals. This information-based encoding technique is quite handy for pinpointing where condition effects manifest in the brain. If you’re interested in unraveling the complex world of brain research and exploring innovative techniques for data analysis, check out the full article!
Information theory is a viable candidate to advance our understanding of how the brain processes information generated in the internal or external environment. With its universal applicability, information theory enables the analysis of complex data sets, is free of requirements about the data structure, and can help infer the underlying brain mechanisms. Information-theoretical metrics such as Entropy or Mutual Information have been highly beneficial for analyzing neurophysiological recordings. However, a direct comparison of the performance of these methods with well-established metrics, such as the t-test, is rare. Here, such a comparison is carried out by evaluating the novel method of Encoded Information with Mutual Information, Gaussian Copula Mutual Information, Neural Frequency Tagging, and t-test. We do so by applying each method to event-related potentials and event-related activity in different frequency bands originating from intracranial electroencephalography recordings of humans and marmoset monkeys. Encoded Information is a novel procedure that assesses the similarity of brain responses across experimental conditions by compressing the respective signals. Such an information-based encoding is attractive whenever one is interested in detecting where in the brain condition effects are present.
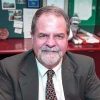
Dr. David Lowemann, M.Sc, Ph.D., is a co-founder of the Institute for the Future of Human Potential, where he leads the charge in pioneering Self-Enhancement Science for the Success of Society. With a keen interest in exploring the untapped potential of the human mind, Dr. Lowemann has dedicated his career to pushing the boundaries of human capabilities and understanding.
Armed with a Master of Science degree and a Ph.D. in his field, Dr. Lowemann has consistently been at the forefront of research and innovation, delving into ways to optimize human performance, cognition, and overall well-being. His work at the Institute revolves around a profound commitment to harnessing cutting-edge science and technology to help individuals lead more fulfilling and intelligent lives.
Dr. Lowemann’s influence extends to the educational platform BetterSmarter.me, where he shares his insights, findings, and personal development strategies with a broader audience. His ongoing mission is shaping the way we perceive and leverage the vast capacities of the human mind, offering invaluable contributions to society’s overall success and collective well-being.