Predicting human motion is like trying to predict a dancer’s next move on the dance floor. While deep learning has made impressive strides in this field, accurately predicting long-term motion and skeletal deformation remain challenging. To address this, a new study presents a two-stage prediction method that combines graph convolutional networks (GCN) with attention mechanisms. In the first stage, a prediction model uses spatial attention graph convolution layers to generate an initial motion sequence based on observed poses. However, this initial pose may not perfectly mimic natural human motion. Thus, the second stage fine-tunes the predicted pose using causally temporal-graph convolution layers. The model is trained by minimizing errors in joint coordinates and bone lengths. Tested on Human3.6m and CMU-MoCap datasets, the two-stage prediction method outperforms previous state-of-the-art methods. While the study acknowledges limitations, its findings pave the way for future breakthroughs in human motion prediction.
Human motion prediction is one of the fundamental studies of computer vision. Much work based on deep learning has shown impressive performance for it in recent years. However, long-term prediction and human skeletal deformation are still challenging tasks for human motion prediction. For accurate prediction, this paper proposes a GCN-based two-stage prediction method. We train a prediction model in the first stage. Using multiple cascaded spatial attention graph convolution layers (SAGCL) to extract features, the prediction model generates an initial motion sequence of future actions based on the observed pose. Since the initial pose generated in the first stage often deviates from natural human body motion, such as a motion sequence in which the length of a bone is changed. So the task of the second stage is to fine-tune the predicted pose and make it closer to natural motion. We present a fine-tuning model including multiple cascaded causally temporal-graph convolution layers (CT-GCL). We apply the spatial coordinate error of joints and bone length error as loss functions to train the fine-tuning model. We validate our model on Human3.6m and CMU-MoCap datasets. Extensive experiments show that the two-stage prediction method outperforms state-of-the-art methods. The limitations of proposed methods are discussed as well, hoping to make a breakthrough in future exploration.
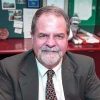
Dr. David Lowemann, M.Sc, Ph.D., is a co-founder of the Institute for the Future of Human Potential, where he leads the charge in pioneering Self-Enhancement Science for the Success of Society. With a keen interest in exploring the untapped potential of the human mind, Dr. Lowemann has dedicated his career to pushing the boundaries of human capabilities and understanding.
Armed with a Master of Science degree and a Ph.D. in his field, Dr. Lowemann has consistently been at the forefront of research and innovation, delving into ways to optimize human performance, cognition, and overall well-being. His work at the Institute revolves around a profound commitment to harnessing cutting-edge science and technology to help individuals lead more fulfilling and intelligent lives.
Dr. Lowemann’s influence extends to the educational platform BetterSmarter.me, where he shares his insights, findings, and personal development strategies with a broader audience. His ongoing mission is shaping the way we perceive and leverage the vast capacities of the human mind, offering invaluable contributions to society’s overall success and collective well-being.