Imagine you’re hosting a talent show and you need to determine the best singer. You decide to use a fancy machine-learning algorithm to analyze the contestants’ brain activity while they imagine singing (mental motor imagery – MI). However, you’re faced with a challenge – there’s no consensus among scientists on how to best analyze the EEG data from MI. In this study, researchers wanted to understand the effects of different preprocessing steps on the accuracy of classifying participant performance using EEG data. They found that the choice of classifier model, such as support-vector machine (SVM) or k-nearest neighbor (KNN), had a significant impact on performance. Interestingly, the researchers discovered that artifact correction and region of interest (ROI) selection did not significantly affect participant performance or classifier performance when using SVM. Additionally, they noticed that the sequence of task execution (starting with resting-state or MI task block) could influence how accurately participant performance was predicted. These findings highlight the importance of considering the classifier model and experiment design when analyzing MI EEG-data. For those eager to dive deeper into this fascinating research, check out the full article!
IntroductionModern consciousness research has developed diagnostic tests to improve the diagnostic accuracy of different states of consciousness via electroencephalography (EEG)-based mental motor imagery (MI), which is still challenging and lacks a consensus on how to best analyse MI EEG-data. An optimally designed and analyzed paradigm must detect command-following in all healthy individuals, before it can be applied in patients, e.g., for the diagnosis of disorders of consciousness (DOC).MethodsWe investigated the effects of two important steps in the raw signal preprocessing on predicting participant performance (F1) and machine-learning classifier performance (area-under-curve, AUC) in eight healthy individuals, that are based solely on MI using high-density EEG (HD-EEG): artifact correction (manual correction with vs. without Independent Component Analysis [ICA]), region of interest (ROI; motor area vs. whole brain), and machine-learning algorithm (support-vector machine [SVM] vs. k-nearest neighbor [KNN]).ResultsResults revealed no significant effects of artifact correction and ROI on predicting participant performance (F1) and classifier performance (AUC) scores (all ps > 0.05) in the SVM classification model. In the KNN model, ROI had a significant influence on the classifier performance [F(1,8.939) = 7.585, p = 0.023]. There was no evidence for artifact correction and ROI selection changing the prediction of participants performance and classifier performance in EEG-based mental MI if using SVM-based classification (71–100% correct classifications across different signal preprocessing methods). The variance in the prediction of participant performance was significantly higher when the experiment started with a resting-state compared to a mental MI task block [X2(1) = 5.849, p = 0.016].DiscussionOverall, we could show that classification is stable across different modes of EEG signal preprocessing when using SVM models. Exploratory analysis gave a hint toward potential effects of the sequence of task execution on the prediction of participant performance, which should be taken into account in future studies.
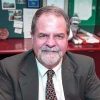
Dr. David Lowemann, M.Sc, Ph.D., is a co-founder of the Institute for the Future of Human Potential, where he leads the charge in pioneering Self-Enhancement Science for the Success of Society. With a keen interest in exploring the untapped potential of the human mind, Dr. Lowemann has dedicated his career to pushing the boundaries of human capabilities and understanding.
Armed with a Master of Science degree and a Ph.D. in his field, Dr. Lowemann has consistently been at the forefront of research and innovation, delving into ways to optimize human performance, cognition, and overall well-being. His work at the Institute revolves around a profound commitment to harnessing cutting-edge science and technology to help individuals lead more fulfilling and intelligent lives.
Dr. Lowemann’s influence extends to the educational platform BetterSmarter.me, where he shares his insights, findings, and personal development strategies with a broader audience. His ongoing mission is shaping the way we perceive and leverage the vast capacities of the human mind, offering invaluable contributions to society’s overall success and collective well-being.