Navigating the vast ocean of information and products on the Internet requires algorithms to guide our choices. These algorithms face a challenge – should they explore uncertain options or exploit known high-rated items? This dilemma, known as the exploration-exploitation trade-off, is influenced by human variability. To better understand this trade-off, researchers have developed a unified model that smoothly transitions between active learning and recommending relevant information. They conducted two experiments to measure the trade-off behavior under different levels of human variability. The results showed that as human variability increases, the trade-off becomes more challenging. However, in a regime of low variability, algorithms balanced in exploration and exploitation can effectively overcome this trade-off.
Human Variability and the Balancing Act of Recommender Systems
Abstract
The enormous scale of the available information and products on the Internet has necessitated the development of algorithms that intermediate between options and human users. These algorithms attempt to provide the user with relevant information. In doing so, the algorithms may incur potential negative consequences stemming from the need to select items about which it is uncertain to obtain information about users versus the need to select items about which it is certain to secure high ratings. This tension is an instance of the exploration–exploitation trade-off in the context of recommender systems. Because humans are in this interaction loop, the long-term trade-off behavior depends on human variability. Our goal is to characterize the trade-off behavior as a function of human variability fundamental to such human–algorithm interaction. To tackle the characterization, we first introduce a unifying model that smoothly transitions between active learning and recommending relevant information. The unifying model gives us access to a continuum of algorithms along the exploration–exploitation trade-off. We then present two experiments to measure the trade-off behavior under two very different levels of human variability. The experimental results inform a thorough simulation study in which we modeled and varied human variability systematically over a wide rage. The main result is that exploration–exploitation trade-off grows in severity as human variability increases, but there exists a regime of low variability where algorithms balanced in exploration and exploitation can largely overcome the trade-off.
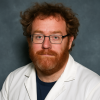
Dr. David Lowemann, M.Sc, Ph.D., is a co-founder of the Institute for the Future of Human Potential, where he leads the charge in pioneering Self-Enhancement Science for the Success of Society. With a keen interest in exploring the untapped potential of the human mind, Dr. Lowemann has dedicated his career to pushing the boundaries of human capabilities and understanding.
Armed with a Master of Science degree and a Ph.D. in his field, Dr. Lowemann has consistently been at the forefront of research and innovation, delving into ways to optimize human performance, cognition, and overall well-being. His work at the Institute revolves around a profound commitment to harnessing cutting-edge science and technology to help individuals lead more fulfilling and intelligent lives.
Dr. Lowemann’s influence extends to the educational platform BetterSmarter.me, where he shares his insights, findings, and personal development strategies with a broader audience. His ongoing mission is shaping the way we perceive and leverage the vast capacities of the human mind, offering invaluable contributions to society’s overall success and collective well-being.