Understanding speech with a cochlear implant (CI) can differ greatly among users due to factors like electrode-nerve interface and neural health conditions. This diversity of performance makes it hard to compare different sound coding strategies through traditional studies. However, computational models offer a controlled environment to assess CI users’ speech performance. In this study, a computational model was used to examine the performance differences between three variants of the HiRes Fidelity 120 (F120) sound coding strategy. The model included a processing stage, an electrode-nerve interface, phenomenological auditory nerve fiber models, and a feature extractor algorithm. The study focused on two experiments: spectral modulation threshold (SMT) and speech reception threshold (SRT). Results showed that neural degeneration had a negative impact on performance with simultaneous stimulation using F120-T, while there was no significant difference in SMT performance. Although the current model needs improvement to accurately predict real-world CI users’ performance, it provides valuable insights into the challenges they face. Future developments in the ANF model and feature extraction algorithms can lead to a more reliable model. To learn more about the research and its implications for cochlear implant users, check out the full article!
Speech understanding in cochlear implant (CI) users presents large intersubject variability that may be related to different aspects of the peripheral auditory system, such as the electrode–nerve interface and neural health conditions. This variability makes it more challenging to proof differences in performance between different CI sound coding strategies in regular clinical studies, nevertheless, computational models can be helpful to assess the speech performance of CI users in an environment where all these physiological aspects can be controlled. In this study, differences in performance between three variants of the HiRes Fidelity 120 (F120) sound coding strategy are studied with a computational model. The computational model consists of (i) a processing stage with the sound coding strategy, (ii) a three-dimensional electrode-nerve interface that accounts for auditory nerve fiber (ANF) degeneration, (iii) a population of phenomenological ANF models, and (iv) a feature extractor algorithm to obtain the internal representation (IR) of the neural activity. As the back-end, the simulation framework for auditory discrimination experiments (FADE) was chosen. Two experiments relevant to speech understanding were performed: one related to spectral modulation threshold (SMT), and the other one related to speech reception threshold (SRT). These experiments included three different neural health conditions (healthy ANFs, and moderate and severe ANF degeneration). The F120 was configured to use sequential stimulation (F120-S), and simultaneous stimulation with two (F120-P) and three (F120-T) simultaneously active channels. Simultaneous stimulation causes electric interaction that smears the spectrotemporal information transmitted to the ANFs, and it has been hypothesized to lead to even worse information transmission in poor neural health conditions. In general, worse neural health conditions led to worse predicted performance; nevertheless, the detriment was small compared to clinical data. Results in SRT experiments indicated that performance with simultaneous stimulation, especially F120-T, were more affected by neural degeneration than with sequential stimulation. Results in SMT experiments showed no significant difference in performance. Although the proposed model in its current state is able to perform SMT and SRT experiments, it is not reliable to predict real CI users’ performance yet. Nevertheless, improvements related to the ANF model, feature extraction, and predictor algorithm are discussed.
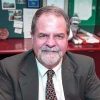
Dr. David Lowemann, M.Sc, Ph.D., is a co-founder of the Institute for the Future of Human Potential, where he leads the charge in pioneering Self-Enhancement Science for the Success of Society. With a keen interest in exploring the untapped potential of the human mind, Dr. Lowemann has dedicated his career to pushing the boundaries of human capabilities and understanding.
Armed with a Master of Science degree and a Ph.D. in his field, Dr. Lowemann has consistently been at the forefront of research and innovation, delving into ways to optimize human performance, cognition, and overall well-being. His work at the Institute revolves around a profound commitment to harnessing cutting-edge science and technology to help individuals lead more fulfilling and intelligent lives.
Dr. Lowemann’s influence extends to the educational platform BetterSmarter.me, where he shares his insights, findings, and personal development strategies with a broader audience. His ongoing mission is shaping the way we perceive and leverage the vast capacities of the human mind, offering invaluable contributions to society’s overall success and collective well-being.