Just like detectives use different clues to solve a crime, scientists are using brain imaging and genetics to unravel the mysteries of Alzheimer’s disease. A new study uses a method called hypergraph-based netNMf to associate brain imaging phenotypes (representations of brain structures) and genetic risk factors in Alzheimer’s and mild cognitive impairment (MCI) patients. By integrating structural magnetic resonance imaging (MRI) data with gene expression profiles, the researchers were able to identify key genes and regions of interest (ROIs) involved in the development and progression of these conditions. This approach relies on the idea that genes and brain structures are interconnected in a complex network, just like puzzle pieces fitting together to reveal the bigger picture. Using this information, the scientists constructed diagnostic models to predict Alzheimer’s disease and MCI. The results showed promising accuracy rates, with an area under the curve (AUC) of 0.8 for AD and 0.797 for MCI. This research opens up new avenues for early diagnosis and potential treatment strategies for neurodegenerative diseases like Alzheimer’s. For more information on this exciting study, be sure to read the full article!
AbstractAlzheimer’s disease (AD) is a severe neurodegenerative disease for which there is currently no effective treatment. Mild cognitive impairment (MCI) is an early disease that may progress to AD. The effective diagnosis of AD and MCI in the early stage has important clinical significance.MethodsTo this end, this paper proposed a hypergraph-based netNMF (HG-netNMF) algorithm for integrating structural magnetic resonance imaging (sMRI) of AD and MCI with corresponding gene expression profiles.ResultsHypergraph regularization assumes that regions of interest (ROIs) and genes were located on a non-linear low-dimensional manifold and can capture the inherent prevalence of two modalities of data and mined high-order correlation features of the two data. Further, this paper used the HG-netNMF algorithm to construct a brain structure connection network and a protein interaction network (PPI) with potential role relationships, mine the risk (ROI) and key genes of both, and conduct a series of bioinformatics analyses.ConclusionFinally, this paper used the risk ROI and key genes of the AD and MCI groups to construct diagnostic models. The AUC of the AD group and MCI group were 0.8 and 0.797, respectively.
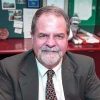
Dr. David Lowemann, M.Sc, Ph.D., is a co-founder of the Institute for the Future of Human Potential, where he leads the charge in pioneering Self-Enhancement Science for the Success of Society. With a keen interest in exploring the untapped potential of the human mind, Dr. Lowemann has dedicated his career to pushing the boundaries of human capabilities and understanding.
Armed with a Master of Science degree and a Ph.D. in his field, Dr. Lowemann has consistently been at the forefront of research and innovation, delving into ways to optimize human performance, cognition, and overall well-being. His work at the Institute revolves around a profound commitment to harnessing cutting-edge science and technology to help individuals lead more fulfilling and intelligent lives.
Dr. Lowemann’s influence extends to the educational platform BetterSmarter.me, where he shares his insights, findings, and personal development strategies with a broader audience. His ongoing mission is shaping the way we perceive and leverage the vast capacities of the human mind, offering invaluable contributions to society’s overall success and collective well-being.