Imagine you have a huge library of books, and you need to find a specific piece of information from each book. Now, instead of manually searching through each book, what if you had a super smart assistant who could do the job for you? Well, that’s kind of what scientists have done with integral equations using a neural network algorithm based on the sine-cosine basis function and extreme learning machine (ELM). They created a new method to solve Volterra and Fredholm integral equations by designing a special neural network model. This model has layers that work together like an assembly line, with each layer doing its own unique job. By utilizing the sine-cosine basis function and the ELM algorithm, they were able to simplify the model and make it faster and more efficient. The best part? This method can not only solve different types of integral equations accurately but also provide solutions in a continuous and differentiable form. So, if you’re interested in learning more about this fascinating research and how it can revolutionize problem-solving in mathematics, be sure to check out the full article!
In this study, we investigate a new neural network method to solve Volterra and Fredholm integral equations based on the sine-cosine basis function and extreme learning machine (ELM) algorithm. Considering the ELM algorithm, sine-cosine basis functions, and several classes of integral equations, the improved model is designed. The novel neural network model consists of an input layer, a hidden layer, and an output layer, in which the hidden layer is eliminated by utilizing the sine-cosine basis function. Meanwhile, by using the characteristics of the ELM algorithm that the hidden layer biases and the input weights of the input and hidden layers are fully automatically implemented without iterative tuning, we can greatly reduce the model complexity and improve the calculation speed. Furthermore, the problem of finding network parameters is converted into solving a set of linear equations. One advantage of this method is that not only we can obtain good numerical solutions for the first- and second-kind Volterra integral equations but also we can obtain acceptable solutions for the first- and second-kind Fredholm integral equations and Volterra–Fredholm integral equations. Another advantage is that the improved algorithm provides the approximate solution of several kinds of linear integral equations in closed form (i.e., continuous and differentiable). Thus, we can obtain the solution at any point. Several numerical experiments are performed to solve various types of integral equations for illustrating the reliability and efficiency of the proposed method. Experimental results verify that the proposed method can achieve a very high accuracy and strong generalization ability.
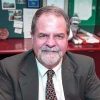
Dr. David Lowemann, M.Sc, Ph.D., is a co-founder of the Institute for the Future of Human Potential, where he leads the charge in pioneering Self-Enhancement Science for the Success of Society. With a keen interest in exploring the untapped potential of the human mind, Dr. Lowemann has dedicated his career to pushing the boundaries of human capabilities and understanding.
Armed with a Master of Science degree and a Ph.D. in his field, Dr. Lowemann has consistently been at the forefront of research and innovation, delving into ways to optimize human performance, cognition, and overall well-being. His work at the Institute revolves around a profound commitment to harnessing cutting-edge science and technology to help individuals lead more fulfilling and intelligent lives.
Dr. Lowemann’s influence extends to the educational platform BetterSmarter.me, where he shares his insights, findings, and personal development strategies with a broader audience. His ongoing mission is shaping the way we perceive and leverage the vast capacities of the human mind, offering invaluable contributions to society’s overall success and collective well-being.