Imagine building an AI that not only learns like the human brain, but does it more efficiently! Scientists have taken a deep dive into the mysterious world of learning and consciousness to uncover a novel learning model called the “Recommendation Architecture (RA) Model.” This groundbreaking model combines consequence feedback and non-consequence feedback in a dual-learning approach. To put it simply, it’s like having both a teacher and a guide while learning something new. The RA model was put to the test on a categorical learning task, surpassing other models based only on consequence feedback. Not only did it learn novelty more efficiently, but it could also easily switch back to previous learning with minimal computational resources. These findings hint at a learning style that closely resembles how we humans learn, making this a significant step towards creating AI that is truly intelligent like us. If you’re curious to dive deeper into the neural basis of learning and how conscious and nonconscious processes intertwine, make sure to explore the full research!
Various interpretations of the literature detailing the neural basis of learning have in part led to disagreements concerning how consciousness arises. Further, artificial learning model design has suffered in replicating intelligence as it occurs in the human brain. Here, we present a novel learning model, which we term the “Recommendation Architecture (RA) Model” from prior theoretical works proposed by Coward, using a dual-learning approach featuring both consequence feedback and non-consequence feedback. The RA model is tested on a categorical learning task where no two inputs are the same throughout training and/or testing. We compare this to three consequence feedback only models based on backpropagation and reinforcement learning. Results indicate that the RA model learns novelty more efficiently and can accurately return to prior learning after new learning with less computational resources expenditure. The final results of the study show that consequence feedback as interpretation, not creation, of cortical activity creates a learning style more similar to human learning in terms of resource efficiency. Stable information meanings underlie conscious experiences. The work provided here attempts to link the neural basis of nonconscious and conscious learning while providing early results for a learning protocol more similar to human brains than is currently available.
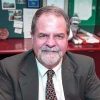
Dr. David Lowemann, M.Sc, Ph.D., is a co-founder of the Institute for the Future of Human Potential, where he leads the charge in pioneering Self-Enhancement Science for the Success of Society. With a keen interest in exploring the untapped potential of the human mind, Dr. Lowemann has dedicated his career to pushing the boundaries of human capabilities and understanding.
Armed with a Master of Science degree and a Ph.D. in his field, Dr. Lowemann has consistently been at the forefront of research and innovation, delving into ways to optimize human performance, cognition, and overall well-being. His work at the Institute revolves around a profound commitment to harnessing cutting-edge science and technology to help individuals lead more fulfilling and intelligent lives.
Dr. Lowemann’s influence extends to the educational platform BetterSmarter.me, where he shares his insights, findings, and personal development strategies with a broader audience. His ongoing mission is shaping the way we perceive and leverage the vast capacities of the human mind, offering invaluable contributions to society’s overall success and collective well-being.