Imagine you’re a detective analyzing clues to solve a mysterious case. In this study, scientists took a similar approach but traded in magnifying glasses for Convolutional Neural Networks (CNNs) to investigate perfusion in patients with acute ischemic stroke. The CNN was trained on pre-processed CT perfusion data, generating informative maps that revealed the extent of blood flow abnormalities. By comparing these CNN-derived maps to ground truth maps created using a deconvolution algorithm, the researchers found a strong agreement between the two methods. The CNN-generated maps not only accurately identified areas of infarct core and hypo-perfused regions, but also significantly reduced the volume of data required for analysis. This breakthrough has exciting implications for the development of novel, low-dose perfusion protocols that could minimize radiation exposure for patients. If you’re curious about the potential of machine learning in stroke research, take a closer look at the underlying study!
ObjectiveIn this study, we investigate whether a Convolutional Neural Network (CNN) can generate informative parametric maps from the pre-processed CT perfusion data in patients with acute ischemic stroke in a clinical setting.MethodsThe CNN training was performed on a subset of 100 pre-processed perfusion CT dataset, while 15 samples were kept for testing. All the data used for the training/testing of the network and for generating ground truth (GT) maps, using a state-of-the-art deconvolution algorithm, were previously pre-processed using a pipeline for motion correction and filtering. Threefold cross validation had been used to estimate the performance of the model on unseen data, reporting Mean Squared Error (MSE). Maps accuracy had been checked through manual segmentation of infarct core and total hypo-perfused regions on both CNN-derived and GT maps. Concordance among segmented lesions was assessed using the Dice Similarity Coefficient (DSC). Correlation and agreement among different perfusion analysis methods were evaluated using mean absolute volume differences, Pearson correlation coefficients, Bland-Altman analysis, and coefficient of repeatability across lesion volumes.ResultsThe MSE was very low for two out of three maps, and low in the remaining map, showing good generalizability. Mean Dice scores from two different raters and the GT maps ranged from 0.80 to 0.87. Inter-rater concordance was high, and a strong correlation was found between lesion volumes of CNN maps and GT maps (0.99, 0.98, respectively).ConclusionThe agreement between our CNN-based perfusion maps and the state-of-the-art deconvolution-algorithm perfusion analysis maps, highlights the potential of machine learning methods applied to perfusion analysis. CNN approaches can reduce the volume of data required by deconvolution algorithms to estimate the ischemic core, and thus might allow the development of novel perfusion protocols with lower radiation dose deployed to the patient.
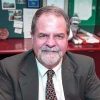
Dr. David Lowemann, M.Sc, Ph.D., is a co-founder of the Institute for the Future of Human Potential, where he leads the charge in pioneering Self-Enhancement Science for the Success of Society. With a keen interest in exploring the untapped potential of the human mind, Dr. Lowemann has dedicated his career to pushing the boundaries of human capabilities and understanding.
Armed with a Master of Science degree and a Ph.D. in his field, Dr. Lowemann has consistently been at the forefront of research and innovation, delving into ways to optimize human performance, cognition, and overall well-being. His work at the Institute revolves around a profound commitment to harnessing cutting-edge science and technology to help individuals lead more fulfilling and intelligent lives.
Dr. Lowemann’s influence extends to the educational platform BetterSmarter.me, where he shares his insights, findings, and personal development strategies with a broader audience. His ongoing mission is shaping the way we perceive and leverage the vast capacities of the human mind, offering invaluable contributions to society’s overall success and collective well-being.