Imagine you’re trying to understand a story without words. Can you tell if the characters are happy, sad, or angry? That’s what researchers are exploring with visual representations. In this study, they compared humans and machines (specifically deep neural networks) to see if both could recognize negation in images. They collected data on how humans interpreted captions of images and found that humans were able to identify certain images as expressing negation. By analyzing these findings, they tested whether humans and machines could classify new images as expressing negation. The results showed that humans were somewhat successful, while the machine learning model struggled to perform at the same level. This suggests that humans have a special ability to recognize negation in visual representations, possibly due to our background knowledge and context. Understanding these differences between human and machine performance can offer insights into human cognitive abilities and guide the development of more human-like artificial intelligence systems. Ready to dive deeper into understanding how we interpret visuals? Check out the research!
Abstract
There is a widely held view that visual representations (images) do not depict negation, for example, as expressed by the sentence, “the train is not coming.” The present study focuses on the real-world visual representations of photographs and comic (manga) illustrations and empirically challenges the question of whether humans and machines, that is, modern deep neural networks, can recognize visual representations as expressing negation. By collecting data on the captions humans gave to images and analyzing the occurrences of negation phrases, we show some evidence that humans recognize certain images as expressing negation. Furthermore, based on this finding, we examined whether or not humans and machines can classify novel images as expressing negation. The humans were able to correctly classify images to some extent, as expected from the analysis of the image captions. On the other hand, the machine learning model of image processing was only able to perform this classification at about the chance level, not at the same level of performance as the human. Based on these results, we discuss what makes humans capable of recognizing negation in visual representations, highlighting the role of the background commonsense knowledge that humans can exploit. Comparing human and machine learning performances suggests new ways to understand human cognitive abilities and to build artificial intelligence systems with more human-like abilities to understand logical concepts.
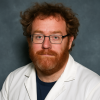
Dr. David Lowemann, M.Sc, Ph.D., is a co-founder of the Institute for the Future of Human Potential, where he leads the charge in pioneering Self-Enhancement Science for the Success of Society. With a keen interest in exploring the untapped potential of the human mind, Dr. Lowemann has dedicated his career to pushing the boundaries of human capabilities and understanding.
Armed with a Master of Science degree and a Ph.D. in his field, Dr. Lowemann has consistently been at the forefront of research and innovation, delving into ways to optimize human performance, cognition, and overall well-being. His work at the Institute revolves around a profound commitment to harnessing cutting-edge science and technology to help individuals lead more fulfilling and intelligent lives.
Dr. Lowemann’s influence extends to the educational platform BetterSmarter.me, where he shares his insights, findings, and personal development strategies with a broader audience. His ongoing mission is shaping the way we perceive and leverage the vast capacities of the human mind, offering invaluable contributions to society’s overall success and collective well-being.