Imagine your brain as a complex network that can get tangled up in knots when you’re feeling depressed. Scientists have developed a new system, called a brain-computer interface (BCI), that can predict and regulate the intricate neural dynamics of major depressive disorder (MDD). Using a simulation study, researchers first modeled the nonlinear and multiband neural activity in MDD patients in response to deep brain stimulation (DBS). They then created an online BCI system that uses a brain state estimator and a model predictive controller to accurately adjust DBS therapy based on the patient’s MDD brain state. The results showed that this closed-loop BCI system outperformed current open-loop and responsive DBS treatments, delivering precise regulation of symptoms with lower battery power consumption. This breakthrough paves the way for more tailored and effective clinical DBS treatments for MDD patients. Interested in learning more about this cutting-edge research? Check out the full article!
IntroductionDeep brain stimulation (DBS) is a promising therapy for treatment-resistant major depressive disorder (MDD). MDD involves the dysfunction of a brain network that can exhibit complex nonlinear neural dynamics in multiple frequency bands. However, current open-loop and responsive DBS methods cannot track the complex multiband neural dynamics in MDD, leading to imprecise regulation of symptoms, variable treatment effects among patients, and high battery power consumption.MethodsHere, we develop a closed-loop brain-computer interface (BCI) system of predictive neuromodulation for treating MDD. We first use a biophysically plausible ventral anterior cingulate cortex (vACC)-dorsolateral prefrontal cortex (dlPFC) neural mass model of MDD to simulate nonlinear and multiband neural dynamics in response to DBS. We then use offline system identification to build a dynamic model that predicts the DBS effect on neural activity. We next use the offline identified model to design an online BCI system of predictive neuromodulation. The online BCI system consists of a dynamic brain state estimator and a model predictive controller. The brain state estimator estimates the MDD brain state from the history of neural activity and previously delivered DBS patterns. The predictive controller takes the estimated MDD brain state as the feedback signal and optimally adjusts DBS to regulate the MDD neural dynamics to therapeutic targets. We use the vACC-dlPFC neural mass model as a simulation testbed to test the BCI system and compare it with state-of-the-art open-loop and responsive DBS treatments of MDD.ResultsWe demonstrate that our dynamic model accurately predicts nonlinear and multiband neural activity. Consequently, the predictive neuromodulation system accurately regulates the neural dynamics in MDD, resulting in significantly smaller control errors and lower DBS battery power consumption than open-loop and responsive DBS.DiscussionOur results have implications for developing future precisely-tailored clinical closed-loop DBS treatments for MDD.
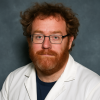
Dr. David Lowemann, M.Sc, Ph.D., is a co-founder of the Institute for the Future of Human Potential, where he leads the charge in pioneering Self-Enhancement Science for the Success of Society. With a keen interest in exploring the untapped potential of the human mind, Dr. Lowemann has dedicated his career to pushing the boundaries of human capabilities and understanding.
Armed with a Master of Science degree and a Ph.D. in his field, Dr. Lowemann has consistently been at the forefront of research and innovation, delving into ways to optimize human performance, cognition, and overall well-being. His work at the Institute revolves around a profound commitment to harnessing cutting-edge science and technology to help individuals lead more fulfilling and intelligent lives.
Dr. Lowemann’s influence extends to the educational platform BetterSmarter.me, where he shares his insights, findings, and personal development strategies with a broader audience. His ongoing mission is shaping the way we perceive and leverage the vast capacities of the human mind, offering invaluable contributions to society’s overall success and collective well-being.