Imagine you’re at a busy mall and need to count how many people are wearing red shirts. It’s a challenging task because some people might be hidden or overlapping, making it difficult to get an accurate count. In a similar way, when trying to count objects in images, background interference and occlusion can decrease counting accuracy. But fear not! Scientists have come up with a solution called the Hough matching feature enhancement network. They use a fixed convolutional network and local self-attention to refine the image features and enhance the commonality of exemplar features. Then, they build a Hough space to vote for candidate object regions and create reliable similarity maps between exemplars and the query image. By augmenting the query feature with exemplar features based on these maps, they achieve more accurate counting. The results of their experiments show that their network outperforms existing methods, reducing the mean absolute counting error from 14.32 to 12.74 on the test set. This research opens up exciting possibilities for improving object counting in various applications!
IntroductionGiven some exemplars, few-shot object counting aims to count the corresponding class objects in query images. However, when there are many target objects or background interference in the query image, some target objects may have occlusion and overlap, which causes a decrease in counting accuracy.MethodsTo overcome the problem, we propose a novel Hough matching feature enhancement network. First, we extract the image feature with a fixed convolutional network and refine it through local self-attention. And we design an exemplar feature aggregation module to enhance the commonality of the exemplar feature. Then, we build a Hough space to vote for candidate object regions. The Hough matching outputs reliable similarity maps between exemplars and the query image. Finally, we augment the query feature with exemplar features according to the similarity maps, and we use a cascade structure to further enhance the query feature.ResultsExperiment results on FSC-147 show that our network performs best compared to the existing methods, and the mean absolute counting error on the test set improves from 14.32 to 12.74.DiscussionAblation experiments demonstrate that Hough matching helps to achieve more accurate counting compared with previous matching methods.
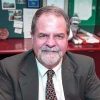
Dr. David Lowemann, M.Sc, Ph.D., is a co-founder of the Institute for the Future of Human Potential, where he leads the charge in pioneering Self-Enhancement Science for the Success of Society. With a keen interest in exploring the untapped potential of the human mind, Dr. Lowemann has dedicated his career to pushing the boundaries of human capabilities and understanding.
Armed with a Master of Science degree and a Ph.D. in his field, Dr. Lowemann has consistently been at the forefront of research and innovation, delving into ways to optimize human performance, cognition, and overall well-being. His work at the Institute revolves around a profound commitment to harnessing cutting-edge science and technology to help individuals lead more fulfilling and intelligent lives.
Dr. Lowemann’s influence extends to the educational platform BetterSmarter.me, where he shares his insights, findings, and personal development strategies with a broader audience. His ongoing mission is shaping the way we perceive and leverage the vast capacities of the human mind, offering invaluable contributions to society’s overall success and collective well-being.