Imagine a performance where sparse coding, computational neuroscience, and information theory come together harmoniously to create BitBrain and Sparse Binary Coincidence (SBC) memories! These innovative tools enable speedy learning and robust inference for brain-like neuromorphic architectures. Inspired by the intricate melodies of a symphony, the SBC memory stores coincidences between detected features in a training set and uses them to identify the class of a new test example. Multiple SBC memories can be combined in a BitBrain, expanding the range of contributing feature coincidences. With excellent classification performance on benchmarks like MNIST and EMNIST, BitBrain achieves accuracy comparable to deep networks but with fewer parameters and lower training costs. Just like a maestro conducting a flawless performance, BitBrain is designed for efficiently training and inferring on conventional as well as neuromorphic architectures. It offers a unique blend of single-pass, single-shot, and continuous supervised learning, making it perfect for edge computing and IoT applications. So grab your baton and dive into the groundbreaking research showcased in the full article!
We present an innovative working mechanism (the SBC memory) and surrounding infrastructure (BitBrain) based upon a novel synthesis of ideas from sparse coding, computational neuroscience and information theory that enables fast and adaptive learning and accurate, robust inference. The mechanism is designed to be implemented efficiently on current and future neuromorphic devices as well as on more conventional CPU and memory architectures. An example implementation on the SpiNNaker neuromorphic platform has been developed and initial results are presented. The SBC memory stores coincidences between features detected in class examples in a training set, and infers the class of a previously unseen test example by identifying the class with which it shares the highest number of feature coincidences. A number of SBC memories may be combined in a BitBrain to increase the diversity of the contributing feature coincidences. The resulting inference mechanism is shown to have excellent classification performance on benchmarks such as MNIST and EMNIST, achieving classification accuracy with single-pass learning approaching that of state-of-the-art deep networks with much larger tuneable parameter spaces and much higher training costs. It can also be made very robust to noise. BitBrain is designed to be very efficient in training and inference on both conventional and neuromorphic architectures. It provides a unique combination of single-pass, single-shot and continuous supervised learning; following a very simple unsupervised phase. Accurate classification inference that is very robust against imperfect inputs has been demonstrated. These contributions make it uniquely well-suited for edge and IoT applications.
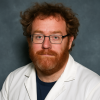
Dr. David Lowemann, M.Sc, Ph.D., is a co-founder of the Institute for the Future of Human Potential, where he leads the charge in pioneering Self-Enhancement Science for the Success of Society. With a keen interest in exploring the untapped potential of the human mind, Dr. Lowemann has dedicated his career to pushing the boundaries of human capabilities and understanding.
Armed with a Master of Science degree and a Ph.D. in his field, Dr. Lowemann has consistently been at the forefront of research and innovation, delving into ways to optimize human performance, cognition, and overall well-being. His work at the Institute revolves around a profound commitment to harnessing cutting-edge science and technology to help individuals lead more fulfilling and intelligent lives.
Dr. Lowemann’s influence extends to the educational platform BetterSmarter.me, where he shares his insights, findings, and personal development strategies with a broader audience. His ongoing mission is shaping the way we perceive and leverage the vast capacities of the human mind, offering invaluable contributions to society’s overall success and collective well-being.