Imagine you’re baking a cake and you want to make sure it turns out just right. You have a recipe, but you also have some knowledge and experience from previous baking experiments. Bayesian hierarchical models in psychology are like using that prior knowledge in your recipe. They combine what we already know with the new data we collect to give us a more accurate result. In this article, the authors explain how they used Bayesian models to analyze psychophysical data from tactile discrimination experiments. By incorporating prior knowledge from the literature and previous experiments into the model, they were able to reduce uncertainty and estimate the effects of experimental variables. This Bayesian approach allowed them to create probability distributions that represent both the uncertainties between and within participants, providing valuable insights into the psychometric functions. The authors used Just Another Gibbs Sampler (JAGS) software to implement their models and R with the rjags package for data analysis. If you’re interested in delving into the details of these powerful Bayesian models for analyzing psychometric functions, check out the full article!
Our previous articles demonstrated how to analyze psychophysical data from a group of participants using generalized linear mixed models (GLMM) and two-level methods. The aim of this article is to revisit hierarchical models in a Bayesian framework. Bayesian models have been previously discussed for the analysis of psychometric functions although this approach is still seldom applied. The main advantage of using Bayesian models is that if the prior is informative, the uncertainty of the parameters is reduced through the combination of prior knowledge and the experimental data. Here, we evaluate uncertainties between and within participants through posterior distributions. To demonstrate the Bayesian approach, we re-analyzed data from two of our previous studies on the tactile discrimination of speed. We considered different methods to include a priori knowledge in the prior distribution, not only from the literature but also from previous experiments. A special type of Bayesian model, the power prior distribution, allowed us to modulate the weight of the prior, constructed from a first set of data, and use it to fit a second one. Bayesian models estimated the probability distributions of the parameters of interest that convey information about the effects of the experimental variables, their uncertainty, and the reliability of individual participants. We implemented these models using the software Just Another Gibbs Sampler (JAGS) that we interfaced with R with the package rjags. The Bayesian hierarchical model will provide a promising and powerful method for the analysis of psychometric functions in psychophysical experiments.
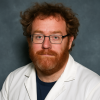
Dr. David Lowemann, M.Sc, Ph.D., is a co-founder of the Institute for the Future of Human Potential, where he leads the charge in pioneering Self-Enhancement Science for the Success of Society. With a keen interest in exploring the untapped potential of the human mind, Dr. Lowemann has dedicated his career to pushing the boundaries of human capabilities and understanding.
Armed with a Master of Science degree and a Ph.D. in his field, Dr. Lowemann has consistently been at the forefront of research and innovation, delving into ways to optimize human performance, cognition, and overall well-being. His work at the Institute revolves around a profound commitment to harnessing cutting-edge science and technology to help individuals lead more fulfilling and intelligent lives.
Dr. Lowemann’s influence extends to the educational platform BetterSmarter.me, where he shares his insights, findings, and personal development strategies with a broader audience. His ongoing mission is shaping the way we perceive and leverage the vast capacities of the human mind, offering invaluable contributions to society’s overall success and collective well-being.