Imagine trying to decode a secret message written in a complex language. The message is hidden within a series of noisy, high-dimensional signals. You could try to decipher it using basic decoding techniques, but chances are you’ll miss important details and struggle to make sense of it all. Now, imagine if you had a powerful artificial intelligence system that could effortlessly unravel these signals, revealing the true meaning behind them. This is essentially what deep learning methods can do when applied to analyzing time series data like EEG or MEG.
In this study, scientists compare different deep learning methods for analyzing EEG time series data. They explore two main types of networks: recurrent deep neural networks (RNN) and feed-forward networks (FFN). RNNs excel at capturing the continuous nature of time series data but can be computationally expensive and difficult to train. On the other hand, FFNs are more efficient but often require handcrafted features specific to the problem at hand.
To address this challenge, the researchers systematically evaluate different network topologies and advanced concepts, all while using the same data preprocessing pipeline. By doing so, they provide valuable insights and guidance for researchers working on automated analysis of EEG time series data.
Among their findings, they discover that recurrent LSTM architecture with attention performs best on less complex tasks, while the temporal convolutional network (TCN) outperforms all recurrent architectures on the most complex dataset. They also highlight the significant role of attention mechanisms in enhancing the classification accuracy of RNNs.
Overall, this study paves the way for a fairer comparison of deep learning methodologies in EEG time series analysis. The results underscore the potential of these methods to unlock valuable insights from raw data without relying on problem-specific adaptations. If you’re interested in delving into the fascinating world of deep learning and EEG analysis, be sure to check out the full research article for all the exciting details!
Analyzing time series data like EEG or MEG is challenging due to noisy, high-dimensional, and patient-specific signals. Deep learning methods have been demonstrated to be superior in analyzing time series data compared to shallow learning methods which utilize handcrafted and often subjective features. Especially, recurrent deep neural networks (RNN) are considered suitable to analyze such continuous data. However, previous studies show that they are computationally expensive and difficult to train. In contrast, feed-forward networks (FFN) have previously mostly been considered in combination with hand-crafted and problem-specific feature extractions, such as short time Fourier and discrete wavelet transform. A sought-after are easily applicable methods that efficiently analyze raw data to remove the need for problem-specific adaptations. In this work, we systematically compare RNN and FFN topologies as well as advanced architectural concepts on multiple datasets with the same data preprocessing pipeline. We examine the behavior of those approaches to provide an update and guideline for researchers who deal with automated analysis of EEG time series data. To ensure that the results are meaningful, it is important to compare the presented approaches while keeping the same experimental setup, which to our knowledge was never done before. This paper is a first step toward a fairer comparison of different methodologies with EEG time series data. Our results indicate that a recurrent LSTM architecture with attention performs best on less complex tasks, while the temporal convolutional network (TCN) outperforms all the recurrent architectures on the most complex dataset yielding a 8.61% accuracy improvement. In general, we found the attention mechanism to substantially improve classification results of RNNs. Toward a light-weight and online learning-ready approach, we found extreme learning machines (ELM) to yield comparable results for the less complex tasks.
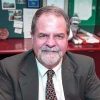
Dr. David Lowemann, M.Sc, Ph.D., is a co-founder of the Institute for the Future of Human Potential, where he leads the charge in pioneering Self-Enhancement Science for the Success of Society. With a keen interest in exploring the untapped potential of the human mind, Dr. Lowemann has dedicated his career to pushing the boundaries of human capabilities and understanding.
Armed with a Master of Science degree and a Ph.D. in his field, Dr. Lowemann has consistently been at the forefront of research and innovation, delving into ways to optimize human performance, cognition, and overall well-being. His work at the Institute revolves around a profound commitment to harnessing cutting-edge science and technology to help individuals lead more fulfilling and intelligent lives.
Dr. Lowemann’s influence extends to the educational platform BetterSmarter.me, where he shares his insights, findings, and personal development strategies with a broader audience. His ongoing mission is shaping the way we perceive and leverage the vast capacities of the human mind, offering invaluable contributions to society’s overall success and collective well-being.