Meltblown nonwoven materials, known for their outstanding filtration performance, are like the superheroes of the textile world. Think of them as the Iron Man suits that shield us from harmful particles. The research on improving their performance through the intercalation meltblown preparation process is an exciting challenge, akin to engineering a state-of-the-art Power Suit. In this study, scientists collected data on intercalated and unintercalated meltblown materials and used statistical analysis to develop a prediction model for these materials under different process parameters. By examining structural variables like thickness, porosity, and compressive resilience, they uncovered how these factors affect product performance, such as filtration resistance, efficiency, and air permeability. To further enhance their predictive powers, they utilized a BP neural network model to maximize filtration efficiency. This groundbreaking research opens up new possibilities for regulating and optimizing the performance of meltblown nonwoven materials. So suit up and venture forth into the fascinating world of meltblown materials – your journey awaits!
Meltblown nonwoven materials have gained attention due to their excellent filtration performance. The research on the performance of the intercalation meltblown preparation process is complex and a current research focus in the field of chemical production. Based on data related to intercalated and unintercalated meltblown materials under given process conditions, a product performance prediction model of intercalated meltblown materials was established under different process parameters (receiving distance, hot air velocity). The structural variables (thickness, porosity, and compressive resilience), the change in product performance, and the relationship between structural variables and product performance (filtration resistance, efficiency, air permeability) after intercalation were studied. Multiple regression analysis was used to analyze the structural variables, and evaluation of the regression results were made using R2, MSE, SSR, and SST. A BP neural network prediction model for product performance was established. The BP neural network model was used to find the maximum filtration efficiency. The study provides theoretical support for regulating product performance by solving the maximum filtration efficiency using BP neural network model.
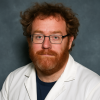
Dr. David Lowemann, M.Sc, Ph.D., is a co-founder of the Institute for the Future of Human Potential, where he leads the charge in pioneering Self-Enhancement Science for the Success of Society. With a keen interest in exploring the untapped potential of the human mind, Dr. Lowemann has dedicated his career to pushing the boundaries of human capabilities and understanding.
Armed with a Master of Science degree and a Ph.D. in his field, Dr. Lowemann has consistently been at the forefront of research and innovation, delving into ways to optimize human performance, cognition, and overall well-being. His work at the Institute revolves around a profound commitment to harnessing cutting-edge science and technology to help individuals lead more fulfilling and intelligent lives.
Dr. Lowemann’s influence extends to the educational platform BetterSmarter.me, where he shares his insights, findings, and personal development strategies with a broader audience. His ongoing mission is shaping the way we perceive and leverage the vast capacities of the human mind, offering invaluable contributions to society’s overall success and collective well-being.